Data Science has emerged as a pivotal field in today’s digital age, revolutionizing industries with its ability to extract valuable insights from vast amounts of data. In India, the significance of data science skills for undergraduates cannot be overstated. As students delve into this domain, engaging in Data Science projects becomes a crucial aspect of their learning journey. These projects offer hands-on experience, allowing students to apply theoretical knowledge to real-world scenarios, and enhancing their problem-solving abilities and analytical skills.
Importance of Data Science Skills for Undergraduates in India
In India, the importance of data science skills for undergraduates is escalating due to the rapid digital transformation across various sectors. By engaging in Data Science projects, students can gain practical experience in data analysis, machine learning, and visualization, which are essential skills in today’s job market. These projects not only reinforce classroom learning but also provide a platform for students to explore diverse applications of data science, preparing them for the dynamic and data-driven industry landscape.
Significance of Data Science Projects for Undergraduates
- Hands-On Learning: Data Science projects offer students the opportunity to work on real datasets, enhancing their practical skills and understanding of data analysis techniques.
- Skill Development: Engaging in projects helps undergraduates develop critical skills such as data wrangling, exploratory data analysis, and machine learning, which are highly sought after by employers.
- Industry Relevance: By working on projects that simulate real-world scenarios, students can bridge the gap between academic knowledge and industry requirements, making them job-ready.
- Career Advancement: Proficiency in data science through projects opens up a plethora of career opportunities in fields like finance, healthcare, e-commerce, and more.
Growing Demand for Data Scientists in India
The demand for data scientists in India is on the rise, driven by the exponential growth of data and the need for actionable insights to drive business decisions. As industries increasingly rely on data-driven strategies, the role of data scientists has become indispensable. Engaging in Data Science projects equips undergraduates with essential skills and experience to meet growing demand. These projects help students excel in the competitive job market by showcasing their expertise.
Data Science Job Market in India
- Industry Integration: Various sectors in India, including IT, healthcare, finance, and e-commerce, are actively recruiting data scientists to leverage data for strategic decision-making.
- Skill Shortage: The demand for data scientists surpasses the current supply, creating ample opportunities for undergraduates with expertise in data science.
- Salary Prospects: Data scientists command competitive salaries in India, reflecting the high demand for professionals who can analyze data effectively and derive actionable insights.
- Career Growth: Data science offers a promising career path with opportunities for advancement and specialization in areas like machine learning, artificial intelligence, and big data analytics.
Benefits of Learning Data Science for Career Growth
Learning data science enhances career prospects and opens diverse opportunities in the evolving tech landscape. Data Science projects are crucial, allowing undergraduates to demonstrate skills, creativity, and problem-solving to employers.
Advantages of Data Science Skills for Career Growth
- Versatility: Data science skills are versatile and applicable across diverse industries, offering professionals the flexibility to explore various career paths.
- Innovation: Data scientists drive innovation by uncovering insights, trends, and patterns that can revolutionize business strategies and decision-making processes.
- Competitive Edge: Proficiency in data science provides a competitive edge in the job market, making individuals highly sought after by top companies.
- Continuous Learning: Data science is a dynamic field that encourages continuous learning and skill development, ensuring professionals stay relevant in a rapidly changing technological landscape.
The journey of learning data science and engaging in Data Science projects is not just about acquiring technical skills; it is about embracing a mindset of curiosity, innovation, and problem-solving that can propel undergraduates toward a successful and fulfilling career in the data-driven world of tomorrow.
Why Should Undergraduates Work on Data Science Projects?
As the demand for data science skills continues to rise in India, undergraduates have a unique opportunity to gain a competitive edge by engaging in Data Science projects. These projects offer numerous benefits that can significantly impact their academic and professional trajectories.
Gain Hands-On Experience with Data Science Tools and Techniques
Data Science projects provide undergraduates with the chance to apply theoretical knowledge to real-world scenarios, allowing them to develop practical skills in data collection, cleaning, analysis, and visualization. By working with various tools and techniques, such as Python, R, SQL, and machine learning algorithms, students can enhance their proficiency and stay up-to-date with industry standards.
Identify Strengths and Weaknesses to Improve Skills
Engaging in Data Science projects helps undergraduates identify their strengths and weaknesses, enabling them to focus on areas that need improvement. This self-assessment process allows students to tailor their learning paths, take targeted courses, and seek mentorship to enhance their skills.
Explore Different Areas of Data Science to Find Interests
Data Science is a vast field with numerous specializations, such as machine learning, natural language processing, computer vision, and time series analysis. By working on diverse projects, undergraduates can explore different areas and discover their interests and passions within the field. This exploration can guide their course selection, internships, and future career paths.
Build an Impressive Portfolio to Stand Out When Applying for Jobs
A well-curated portfolio showcasing successful Data Science projects can significantly enhance an undergraduate’s job prospects. Employers value candidates who have hands-on experience and can demonstrate their ability to solve real-world problems. By including these projects in their resumes and portfolios, undergraduates can differentiate themselves from their peers and increase their chances of landing coveted data science positions.
Boost Confidence and Sense of Accomplishment
Completing Data Science projects can instill a sense of confidence and accomplishment in undergraduates. As they navigate through the challenges of data cleaning, model building, and presenting insights, they develop a growth mindset and learn to overcome obstacles. This experience can boost their self-esteem and motivate them to tackle more complex problems in the future.
Undergraduates who engage in Data Science projects can gain invaluable skills, explore their interests, build impressive portfolios, and boost their confidence. These projects serve as a bridge between academic knowledge and industry requirements, preparing students for successful careers in the dynamic and data-driven world of tomorrow.
Top Data Science Project Ideas for Beginners
Embarking on Data Science projects is a pivotal step for beginners looking to enhance their skills, gain practical experience, and build a strong portfolio. These projects not only provide hands-on learning opportunities but also allow individuals to explore various facets of data science while working on real-world problems.

Fake News Detection
Fake news detection is a critical project that leverages natural language processing and machine learning techniques to distinguish between real and fake news articles. By analyzing text data and identifying patterns indicative of misinformation, this project helps in combating the spread of false information in the digital space.
Chatbot Development
Creating a chatbot is an engaging project that involves natural language processing and artificial intelligence. Chatbots are automated conversational agents that can interact with users, answer queries, and provide assistance. Building a chatbot from scratch allows beginners to delve into the realm of AI-driven communication systems.
Loan Prediction
Loan prediction projects involve predictive modeling to assess the creditworthiness of loan applicants. By analyzing historical data on loan applicants and their repayment behavior, this project aims to predict whether a new applicant is likely to repay the loan. It provides valuable insights for financial institutions in making informed lending decisions.
Credit Card Fraud Detection
Credit card fraud detection projects focus on developing algorithms to identify fraudulent transactions in real-time. By analyzing transaction data and detecting unusual patterns or anomalies, this project helps financial institutions prevent fraudulent activities and protect customers from unauthorized transactions.
Handwritten Digit Recognition
Handwritten digit recognition projects involve image processing and machine learning techniques to recognize and classify handwritten digits. This project is a classic example of pattern recognition and can be implemented using algorithms like convolutional neural networks (CNNs) to achieve high accuracy in digit recognition tasks.
Engaging in these top Data Science projects for beginners offers a valuable learning experience, allowing individuals to apply data science concepts in practical scenarios, hone their skills, and showcase their capabilities to potential employers. By working on projects like fake news detection, chatbot development, loan prediction, credit card fraud detection, and handwritten digit recognition, beginners can lay a solid foundation for a successful career in the dynamic field of data science.
Intermediate Data Science Project Ideas
As you progress in your data science journey, it’s essential to tackle projects that challenge your skills and push you to explore new techniques and tools. Intermediate Data Science projects offer a perfect opportunity to apply your knowledge in real-world scenarios, enhancing your analytical and problem-solving abilities.
Sentiment Analysis
Sentiment analysis projects involve developing algorithms to classify text data as positive, negative, or neutral based on the sentiment expressed. This project is crucial in understanding customer opinions, product reviews, and social media feedback, enabling businesses to make informed decisions.
Movie Recommendation System
Building a movie recommendation system is an engaging project that leverages collaborative filtering and natural language processing techniques. By analyzing user ratings and movie attributes, this project aims to suggest personalized movie recommendations, enhancing the user experience.
Customer Segmentation
Customer segmentation projects involve clustering customers based on their demographic, behavioral, and transactional data. This project helps businesses identify target audiences, tailor marketing strategies, and improve customer satisfaction.
Breast Cancer Classification
Breast cancer classification projects develop predictive models to classify tumors as malignant or benign. These models use medical imaging and patient data for accurate tumor classification. This project contributes to the early detection and treatment of breast cancer, improving patient outcomes.
Tweets Classification
Tweet classification projects involve developing natural language processing algorithms to classify tweets such as spam, news, or opinions. This project is essential for understanding public sentiment, tracking trends, and identifying influencers on social media.
These intermediate Data Science projects offer a platform for individuals to apply their knowledge in real-world scenarios, enhancing their analytical and problem-solving abilities. By working on projects like sentiment analysis, movie recommendation systems, customer segmentation, breast cancer classification, and tweets classification, individuals can develop a strong portfolio, demonstrating their capabilities to potential employers and advancing their careers in the dynamic field of data science.
Advanced-Data Science Project Ideas
As data science continues to evolve, the complexity and scope of projects have also expanded. Advanced data science projects often involve tackling complex problems, working with large datasets, and leveraging cutting-edge techniques. These projects not only showcase your skills but also contribute to solving real-world challenges. Here are some exciting advanced data science project ideas to consider:
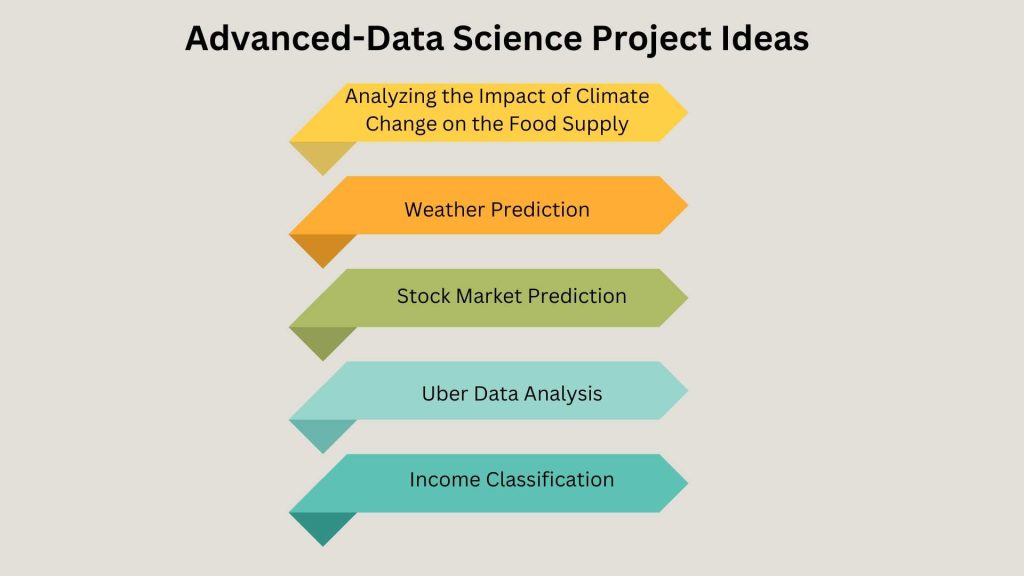
Analyzing the Impact of Climate Change on the Food Supply
- Description: Climate change is a pressing global issue that affects various aspects of our lives, including food security. In this project, you will analyze the impact of climate change on agricultural production, food prices, and food availability.
- Methodology: Collect data from various sources, such as government agencies, research institutions, and international organizations, on factors like temperature, precipitation, crop yields, and food prices.
- Expected Output: A comprehensive analysis and prediction of the impact of climate change on the global food supply, with actionable insights for policymakers and stakeholders.
Weather Prediction
- Description: Accurate weather prediction is crucial for various sectors, including agriculture, transportation, and disaster management. In this project, you will develop a machine learning model to predict weather patterns based on historical data.
- Methodology: Collect weather data from reliable sources, such as weather stations or online APIs, including variables like temperature, humidity, wind speed, and precipitation.
- Expected Output: A highly accurate weather prediction model that can forecast weather conditions for a specific location or region, with potential applications in various industries.
Stock Market Prediction
- Description: Predicting stock market movements is a challenging yet rewarding task in data science. In this project, you will develop a model to forecast stock prices based on historical data and market trends.
- Methodology: Collect stock market data, including stock prices, trading volumes, and relevant economic indicators.
- Expected Output: A stock market prediction model that can forecast stock prices with a high degree of accuracy, potentially generating profitable trading strategies.
Uber Data Analysis
- Description: Uber, a leading ride-sharing company, generates vast amounts of data on rides, drivers, and passengers. In this project, you will analyze Uber’s data to gain insights into the company’s operations and performance.
- Methodology: Collect Uber data from publicly available sources or through web scraping techniques.
- Expected Output: A comprehensive analysis of Uber’s data, providing insights into the company’s operations, performance, and potential areas for improvement.
Income Classification
- Description: Income classification is a crucial task in data science, with applications in fields like marketing, finance, and social welfare. In this project, you will develop a model to classify individuals based on their income level using census data.
- Methodology: Collect census data containing information about individuals, such as age, education, occupation, and income.
- Expected Output: A highly accurate income classification model that can predict an individual’s income level based on their demographic and socioeconomic characteristics, with potential applications in targeted marketing, credit risk assessment, or social welfare programs.
These advanced data science projects cover a wide range of domains and techniques, from climate change analysis to stock market prediction. By working on these projects, you can showcase your skills in data collection, preprocessing, exploratory analysis, machine learning, and visualization. These projects can be valuable additions to your portfolio, demonstrating your ability to tackle complex problems and generate actionable insights from data.
How to Get Started with Data Science Projects
Embarking on data science projects can be a rewarding journey, but it’s essential to approach it systematically to build a strong foundation in this field. Here’s a comprehensive guide to help you kickstart your data science project journey using insights from various sources:
Learn Python and Data Science Fundamentals
- Python Programming: Master the basics of Python, a versatile and widely used programming language in data science. Learn about data structures, control flow, and libraries like NumPy and Pandas for data manipulation.
- Data Science Fundamentals: Understand the core concepts of data science, including data collection, cleaning, exploratory data analysis, and machine learning algorithms. Familiarize yourself with statistical techniques and data visualization tools.
- Statistics and Mathematics: Develop a solid foundation in statistics and mathematics, including probability, regression, and hypothesis testing, which are essential for data analysis and modeling.
Choose a Project Based on Skill Level and Interests
- Beginner-Level Projects: Start with projects like fake news detection, chatbot development, or credit card fraud detection to build your skills and confidence. Choose projects that align with your interests and skill level.
- Intermediate Level Projects: Progress to more challenging projects like weather prediction, stock market prediction, or Uber data analysis. These projects will help you deepen your understanding and apply advanced techniques.
- Advanced Level Projects: Challenge yourself with complex projects like analyzing the impact of climate change on the food supply or income classification. These projects will push your skills to the next level and demonstrate your expertise in data science.
Gather Relevant Datasets
- Identify the Problem: Define the problem you want to solve and the type of data you need to address it. Look for datasets that are relevant to your project goals and can provide valuable insights.
- Source Data: Collect data from various sources, such as online repositories, government databases, or APIs. Ensure that the data is reliable, relevant, and suitable for your analysis.
- Assess Data Quality: Evaluate the quality of the dataset by checking for completeness, accuracy, and consistency. Address any issues like missing values or outliers before proceeding with your analysis.
Clean and Preprocess Data
- Data Cleaning: Handle missing values, duplicates, and inconsistencies in the dataset. Use techniques like imputation, data normalization, and outlier detection to ensure data quality.
- Feature Engineering: Create new features from existing data to improve model performance. Transform categorical variables, scale numerical features, and encode data for machine learning algorithms.
- Data Transformation: Prepare the data for analysis by encoding categorical variables, scaling features, and splitting the dataset into training and testing sets. Ensure that the data is in a format suitable for modeling.
Perform Exploratory Data Analysis
- Visualize Data: Use charts, graphs, and statistical summaries to explore patterns, trends, and relationships in the data. Identify outliers, correlations, and insights that can guide your analysis.
- Statistical Analysis: Calculate descriptive statistics, distributions, and correlations to understand the data better. Use hypothesis testing and statistical tests to validate assumptions and draw meaningful conclusions.
- Data Visualization: Create visualizations that communicate key findings and insights effectively. Use tools like Matplotlib, Seaborn, or Plotly to generate informative and visually appealing plots.
Build and Evaluate Machine Learning Models
- Select Algorithms: Choose appropriate machine learning algorithms based on the nature of the problem and the type of data. Experiment with regression, classification, clustering, or deep learning models to address the project goals.
- Train and Test Models: Split the data into training and testing sets to train the model and evaluate its performance. Use metrics like accuracy, precision, recall, or F1 score to assess model effectiveness.
- Hyperparameter Tuning: Optimize model performance by tuning hyperparameters, adjusting model complexity, and improving generalization. Use techniques like grid search, cross-validation, and ensemble methods to enhance model accuracy.
Present Findings and Insights
- Communicate Results: Prepare a report or presentation that summarizes your findings, methodology, and key insights from the project. Use visualizations, tables, and narratives to convey complex information.
- Share Learnings: Discuss your project with peers, mentors, or the data science community to gather feedback and insights. Present your work at meetups, conferences, or online platforms to showcase your skills and expertise.
- Update Portfolio: Include your data science project in your portfolio, resume, or LinkedIn profile to demonstrate your practical experience and problem-solving abilities to potential employers. Highlight the impact of your project and the value it brings to the organization or domain.
Following these steps and engaging in data science projects can enhance your skills significantly. Actively participating in projects helps build a strong portfolio to showcase your abilities. Positioning yourself for success in data science requires dedication and hands-on experience in the field. Remember to stay curious, keep learning, and embrace challenges as opportunities for growth and development.
Tips for Successful Data Science Projects
Embarking on data science projects requires a strategic approach to ensure success and maximize learning opportunities. Here are essential tips to guide you through your data science project journey, drawing insights from various sources:
Choose an Interesting Problem to Stay Motivated
- Problem Selection: Opt for a problem that piques your interest and aligns with your goals. Choosing a captivating problem will keep you motivated throughout the project and drive your enthusiasm for exploration and discovery.
- Relevance: Ensure that the problem you choose has real-world relevance and practical implications. Solving meaningful problems can enhance your sense of accomplishment and contribute to your professional growth.
- Personalization: Tailor the problem statement to your interests and expertise. Personalizing the project can make the journey more engaging and enjoyable, leading to a more fulfilling learning experience.
Start with a Simple Project and Gradually Increase the Complexity
- Progressive Learning: Begin with a straightforward project to grasp the basics and build a strong foundation. Starting simply allows you to understand fundamental concepts and techniques before tackling more complex challenges.
- Skill Development: As you gain confidence and proficiency, gradually increase the complexity of your projects. Challenge yourself with new methodologies, algorithms, or datasets to expand your skills and knowledge in data science.
- Iterative Approach: Embrace an iterative approach to project development. Break down complex problems into manageable tasks and iterate on your solutions to enhance performance and efficiency.
Document Code and Findings
- Code Documentation: Maintain clear and well-structured documentation for your code. Documenting your code ensures readability, reproducibility, and collaboration with team members or stakeholders.
- Findings Documentation: Record your findings, insights, and methodologies throughout the project. Documenting your discoveries helps in tracking progress, sharing knowledge, and presenting results effectively.
- Version Control: Utilize version control systems like Git to track changes in your codebase and collaborate with others seamlessly. Version control enhances code management, facilitates collaboration, and ensures project integrity.
Seek Feedback and Collaborate with Others
- Feedback Loop: Seek feedback from peers, mentors, or the data science community. Feedback provides valuable insights, alternative perspectives, and constructive criticism that can help improve your project outcomes.
- Collaboration: Collaborate with other data science enthusiasts, professionals, or domain experts. Collaborative projects foster knowledge sharing, skill development, and innovative problem-solving approaches.
- Peer Review: Engage in peer review sessions to receive feedback on your work. Peer reviews can help identify blind spots, errors, or areas for improvement, enhancing the quality and robustness of your project.
Continuously Learn and Improve Skills
- Lifelong Learning: Embrace a mindset of continuous learning and skill development. Stay updated with the latest trends, technologies, and best practices in data science to enhance your expertise and stay competitive.
- Professional Development: Invest in ongoing education, training, and certifications to broaden your skill set and deepen your understanding of data science concepts and methodologies5.
- Practice Makes Perfect: Regularly practice coding, data analysis, and machine learning techniques. Hands-on experience through projects, challenges, and competitions is key to honing your skills and mastering data science.
Incorporating these tips into your data science projects can significantly enhance your learning experience. Improving project outcomes through these strategies helps build a strong foundation in data science. These methods are crucial for establishing a successful career in the dynamic field of data science. Remember, persistence, curiosity, and a willingness to learn are key to mastering data science projects and achieving your goals.
Conclusion
In conclusion, data science skills have become increasingly crucial for undergraduates in India, as the demand for data scientists continues to grow across various industries. Engaging in data science projects not only enhances practical skills but also boosts career prospects and opens doors to exciting opportunities. We encourage undergraduates to start learning data science by exploring online courses, participating in competitions, and working on real-world projects. Through dedication, undergraduates can build a strong foundation in data science. Consistent practice enhances undergraduates’ skills in data science. Willingness to learn propels undergraduates toward success in the dynamic field of data science.
Frequently Asked Questions (FAQs)
1) What are the applications of data science?
Data science has many applications, including predictive analytics, machine learning, data visualization, recommendation systems, fraud detection, and internet search. Various industries utilize data science, including healthcare, finance, e-commerce, transportation, and also gaming.
2) Where is data science used in real life?
Data scientists use data science in real-life scenarios like healthcare for medical image analysis and drug development, in finance for fraud detection and also risk management, in e-commerce for personalized recommendations and targeted advertising, and in transportation for route optimization and also flight delay prediction.
3) What is the current use of data science?
Industries like healthcare, finance, e-commerce, and transportation extensively use data science to drive data-driven decision-making, enhance customer experiences, optimize processes, and improve overall efficiency.
4) What are the applications of data science in society?
Data science is having a significant impact on society, with applications in areas like law enforcement for crime prevention, pandemic response for contact tracing and resource allocation, and driverless vehicles for improved safety and efficiency.
5) Which is the most useful application of data science?
Data science is one of the most useful applications in search engines, where it actively provides the most relevant and personalized search results to users by analyzing vast amounts of data and user behavior.