Target Audience: This article aims that this introduction to data interpretation and communication is designed for a diverse target audience. It likely caters to both students and professionals who need to develop strong data analysis. The content may be particularly relevant for those working in fields like business, healthcare, education, and other data-driven industries where interpreting and effectively communicating insights from data is crucial. Understanding the specific needs and backgrounds of the intended learners will help ensure the material is tailored to maximize its value and impact.
Value Proposition: The comprehensive overview of data interpretation and communication presented in this table of contents promises to deliver significant benefits to learners. By mastering the concepts, methods, and best practices covered, individuals can expect to enhance their decision-making capabilities, problem-solving skills, and overall real-world impact across a variety of applications. The unique combination of theoretical knowledge and practical, hands-on projects sets this introduction apart, equipping learners with a well-rounded skill set that is highly valuable in today’s data-driven landscape.
Key Takeaways: This table of contents highlights the most important elements that learners can expect to take away from this introduction to data interpretation and communication. From understanding the differences between quantitative and qualitative data to exploring various data interpretation techniques like descriptive, inferential, predictive, and prescriptive analysis, the content provides a solid foundation. Additionally, the emphasis on data visualization tools and effective communication strategies ensures that learners develop a comprehensive set of skills to interpret, analyze, and convey data-driven insights effectively. The inclusion of practical, applied learning projects further reinforces the applicability of the concepts covered.
Data Interpretation and Communication: A Quick Introduction
As an engineering student, you are likely familiar with the importance of data in driving innovation, solving complex problems, and optimizing processes. However, simply collecting and analyzing data is not enough – the true power lies in interpreting that data and effectively communicating the insights to stakeholders. This introduction will provide you with a solid foundation in data interpretation and communication, equipping you with practical skills and knowledge that will be invaluable throughout your engineering career.
Data interpretation and communication are essential skills for engineers and professionals across various industries. As the world becomes increasingly data-driven, the ability to effectively interpret, analyze, and communicate insights from data is crucial for making informed decisions, solving complex problems, and driving innovation.
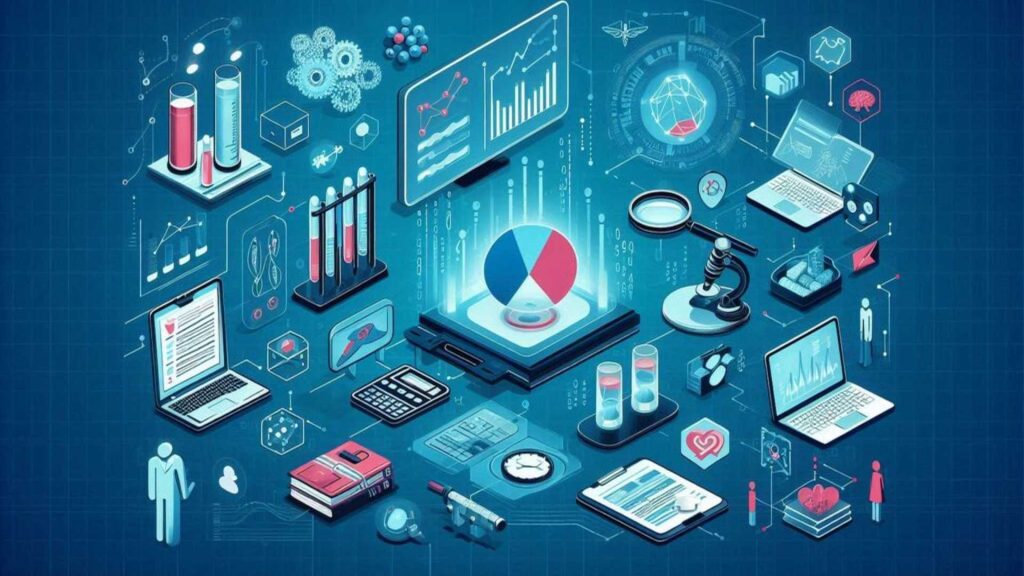
Importance of Data Interpretation
Data interpretation is the process of extracting meaningful insights from raw data by analyzing patterns, trends, and relationships. It involves transforming data into actionable information that can inform decision-making and drive positive outcomes.
The importance of data interpretation cannot be overstated in today’s data-driven world. Here are some key reasons why data interpretation is crucial:
- Informed Decision-Making: By interpreting data effectively, you can make informed decisions based on evidence rather than assumptions. This leads to more accurate and effective strategies that align with your goals and objectives.
- Improved Efficiency: Data interpretation helps identify areas for improvement and optimization. By understanding where inefficiencies lie, you can implement targeted solutions to streamline processes and enhance productivity.
- Competitive Advantage: In a competitive landscape, the ability to extract valuable insights from data can provide a significant advantage. By uncovering trends and opportunities that others may miss, you can differentiate your offerings and stay ahead of the curve.
- Enhanced Customer Experience: Data interpretation enables a deeper understanding of customer behavior, preferences, and pain points. By leveraging these insights, you can tailor your products, services, and marketing efforts to better meet customer needs and improve satisfaction.
- Data-Driven Innovation: Interpreting data can spark new ideas and inspire innovative solutions. By identifying patterns and connections that may not be immediately obvious, you can develop groundbreaking products, services, or processes that disrupt the status quo.
Mastering data interpretation is a valuable skill that can be applied across various industries and domains. Whether you’re working in business, healthcare, education, or scientific research, the ability to extract meaningful insights from data can help you make more informed decisions, drive positive change, and achieve your goals.
Key Concepts and Terminology
Data interpretation involves the analysis and understanding of data to extract meaningful insights and draw conclusions. It encompasses a range of key concepts and terminology that are essential for effective data analysis and decision-making.
Here are some of the key concepts and terminology in data interpretation:
- Variables: Variables are the characteristics or measurements being studied in a dataset. They can be classified as independent (cause) or dependent (effect) variables.
- Data Types: Data types refer to the different categories of data, such as numerical (continuous or discrete), categorical (nominal or ordinal), or textual. Understanding data types is crucial for selecting appropriate analysis techniques.
- Descriptive Statistics: Descriptive statistics summarize and describe the main features of a dataset, such as measures of central tendency (mean, median, mode) and measures of variability (range, variance, standard deviation).
- Inferential Statistics: Inferential statistics involve concluding a population based on a sample of data. This includes techniques such as hypothesis testing, confidence intervals, and regression analysis.
- Correlation: Correlation measures the strength and direction of the linear relationship between two variables. It ranges from -1 to 1, with -1 indicating a perfect negative correlation, 0 indicating no correlation, and 1 indicating a perfect positive correlation.
- Causation: Causation refers to the relationship between two variables where one variable directly causes the other. Establishing causation requires carefully designed experiments or observational studies.
- Confounding Variables: Confounding variables are factors that influence both the independent and dependent variables, potentially distorting the observed relationship between them.
- Outliers: Outliers are data points that are significantly different from the rest of the dataset. They can have a significant impact on the results of data analysis and may need to be addressed or removed.
- Visualization: Data visualization involves the graphical representation of data, such as charts, graphs, and plots. Effective visualization can help communicate complex information clearly and concisely.
Understanding these key concepts and terminology is essential for interpreting data accurately and drawing valid conclusions. By mastering these concepts, you can develop a deeper understanding of data and make more informed decisions based on the insights you uncover.
Before diving into the specifics of data interpretation, it’s essential to understand the fundamental concepts and terminology. We’ll cover topics such as quantitative vs. qualitative data, descriptive and inferential statistics, and the role of data visualization in communicating insights. By establishing a strong conceptual foundation, you’ll be better equipped to tackle more advanced data analysis challenges.
Why Data Interpretation is Crucial
Data interpretation is the process of reviewing data and drawing conclusions from it. It involves analyzing datasets to find meaningful patterns, relationships, and trends. This process is crucial for several reasons:
- Informed Decision-Making: Data interpretation transforms raw data into actionable insights, which are essential for making informed decisions. It allows organizations to base their strategies on evidence rather than intuition.
- Identifying Trends: By interpreting data, organizations can identify trends that might not be immediately obvious. This can include market trends, consumer behavior patterns, or operational inefficiencies.
- Predictive Analysis: Effective data interpretation can help predict future outcomes, enabling proactive measures. For example, interpreting sales data can help forecast future sales and prepare accordingly.
- Problem-Solving: Interpreting data helps in diagnosing problems by uncovering the underlying causes. For example, if a business is experiencing a drop in sales, data interpretation can reveal whether this is due to market conditions, product issues, or other factors.
Impact on Decision-Making
Data interpretation significantly impacts decision-making in various ways:
- Strategic Planning: Organizations can develop more effective strategies by understanding data. For example, a company can adjust its marketing strategy based on consumer response data.
- Operational Efficiency: Data interpretation helps in optimizing operations. For instance, by analyzing production data, a manufacturer can identify bottlenecks and improve efficiency.
- Risk Management: Interpreting data can help identify potential risks and develop mitigation strategies. For example, financial institutions use data interpretation to assess credit risks.
- Customer Insights: Understanding customer data allows businesses to tailor their products and services to meet customer needs better, leading to increased satisfaction and loyalty.
Real-World Examples
- Healthcare: In healthcare, data interpretation is used to improve patient outcomes. For instance, interpreting patient data can help identify the effectiveness of different treatments and tailor personalized treatment plans.
Example: A hospital analyzes patient records to identify patterns in treatment outcomes for heart disease, leading to the development of more effective treatment protocols.
- Retail: Retailers use data interpretation to understand consumer behavior and optimize inventory management.
Example: A retail chain analyzes sales data to determine which products are most popular in different regions, allowing them to stock stores more efficiently.
- Finance: Financial institutions use data interpretation to make investment decisions and manage risks.
Example: A bank uses transaction data to detect fraudulent activity, improve security, and protect customer assets.
- Marketing: Marketers use data interpretation to measure campaign effectiveness and refine their strategies.
Example: A company analyzes social media engagement data to understand which types of content resonate most with their audience, informing future marketing efforts.
Industry Relevance
Data interpretation is relevant across various industries due to its ability to drive efficiency, innovation, and competitive advantage:
- Manufacturing: In manufacturing, interpreting production data helps optimize processes, reduce waste, and improve product quality.
- Education: Educational institutions use data interpretation to enhance learning outcomes by understanding student performance and identifying areas for improvement.
- Energy: The energy sector uses data interpretation to manage resources more effectively, predict demand, and improve sustainability efforts.
- Logistics: Logistics companies rely on data interpretation to optimize routes, reduce costs, and improve delivery times.
By incorporating these elements, students can gain a comprehensive understanding of the importance of data interpretation, its impact on decision-making, and its relevance across various industries. This knowledge will equip them with the skills needed to leverage data effectively in their future careers.
Types of Data in Interpretation
Understanding the types of data used in interpretation is crucial for extracting meaningful insights. Data can be broadly categorized into two types: quantitative data and qualitative data. Each type has its characteristics, methods of analysis, and applications.
Quantitative Data
Quantitative data refers to data that can be measured and expressed numerically. It is often used for statistical analysis and can be used to identify patterns, trends, and correlations. Quantitative data is objective and can be verified through mathematical calculations.
Characteristics of Quantitative Data
- Numerical: Quantitative data consists of numbers and can be quantified.
- Objective: It is based on measurable facts and figures.
- Statistical Analysis: It can be analyzed using statistical methods to conclude.
- Scalable: It can be aggregated and compared across different groups or periods.
Examples of Quantitative Data
- Sales Figures: Monthly sales data of a company.
- Survey Ratings: Customer satisfaction ratings on a scale of 1 to 10.
- Production Quantities: Number of units produced in a factory.
- Website Traffic: Number of visitors to a website per day.
Qualitative Data
Qualitative data refers to non-numerical data that describes qualities or characteristics. It is often used to gain insights into underlying reasons, opinions, and motivations. Qualitative data is subjective and can provide a deeper understanding of a context or phenomenon.
Characteristics of Qualitative Data
- Descriptive: Qualitative data consists of descriptions and narratives.
- Subjective: It is based on personal experiences and interpretations.
- Contextual: It provides context and depth to numerical data.
- Exploratory: It is used to explore ideas and generate hypotheses.
Examples of Qualitative Data
- Customer Feedback: Comments and reviews from customers.
- Interview Transcripts: Transcriptions of interviews with employees or stakeholders.
- Focus Group Discussions: Notes and observations from focus group sessions.
- Social Media Posts: User-generated content and discussions on social media platforms.
Integrating Quantitative and Qualitative Data
Combining quantitative and qualitative data can provide a more comprehensive view of a situation. Quantitative data can highlight trends and patterns, while qualitative data can explain the reasons behind those trends.
Example of Integration
- Customer Satisfaction Analysis:
- Quantitative Data: Average customer satisfaction rating of 8 out of 10.
- Qualitative Data: Customer comments reveal that they appreciate the product quality but find the customer service lacking.
- Visual Representation: A combined bar chart and word cloud showing satisfaction ratings alongside common feedback themes.
Understanding both quantitative and qualitative data is essential for effective data interpretation. By using appropriate methods to analyze and visualize each type of data, students can gain valuable insights and make informed decisions. Combining these data types provides a richer, more nuanced understanding of the data, leading to better outcomes in both academic and professional settings.
Methods of Data Interpretation
Data interpretation involves various methods that help in making sense of collected data. These methods can be categorized into four main types: descriptive analysis, inferential analysis, predictive analysis, and prescriptive analysis. Each method serves a different purpose and is used in different contexts to derive meaningful insights.
Descriptive Analysis
Descriptive analysis is the process of summarizing and organizing data to describe its main characteristics. This type of analysis provides a simple overview of the data without making any predictions or drawing conclusions beyond the data itself.
Key Features
- Summarization: Descriptive analysis provides a summary of the data using measures of central tendency (mean, median, mode) and measures of dispersion (range, variance, standard deviation).
- Visualization: It often involves graphical representation such as charts, graphs, and tables to make the data more understandable.
- Data Presentation: It helps in presenting the data in a way that highlights its essential features.
Examples
- Sales Report: A report showing the average sales per month.
- Customer Demographics: A summary of customer ages, genders, and locations.
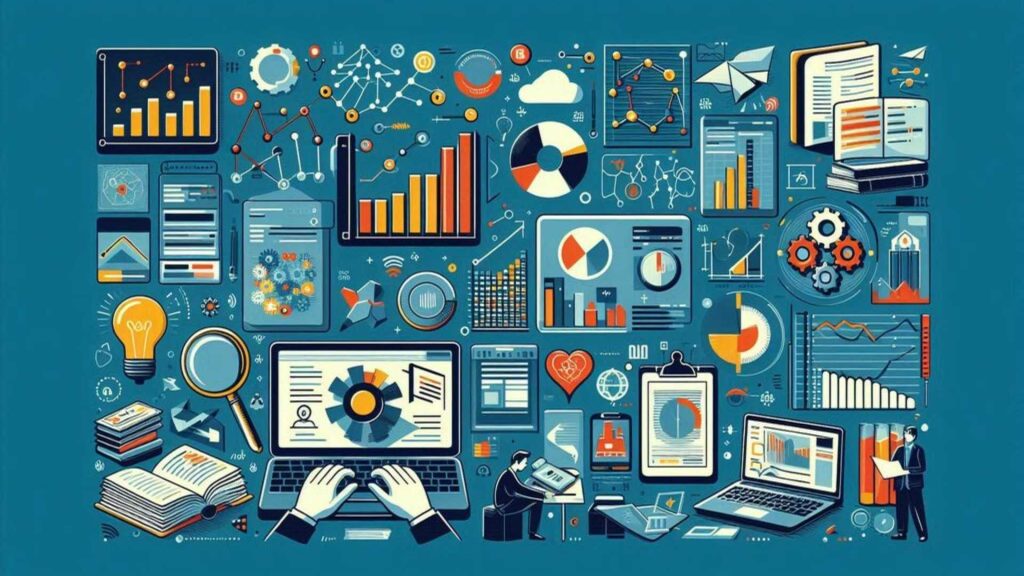
Inferential Analysis
Inferential analysis is used to make predictions or inferences about a population based on a sample of data. It involves hypothesis testing, confidence intervals, and regression analysis to determine relationships between variables.
Key Features
- Hypothesis Testing: Determines if there is enough evidence to support a specific hypothesis about the data.
- Confidence Intervals: Estimates the range within which a population parameter lies with a certain level of confidence.
- Regression Analysis: Identifies relationships between variables and predicts the value of one variable based on another.
Examples
- Medical Study: Testing whether a new drug is more effective than an existing one.
- Market Research: Predicting customer preferences based on a survey sample.
Predictive Analysis
Predictive analysis uses statistical techniques and machine learning algorithms to predict future outcomes based on historical data. It is commonly used in fields such as finance, marketing, and healthcare to forecast trends and behaviors.
Key Features
- Trend Analysis: Identifies patterns and trends in historical data to make future predictions.
- Machine Learning Models: Uses algorithms to predict future events based on past data.
- Forecasting: Estimates future values of a variable based on its historical trends.
Examples
- Stock Market: Predicting stock prices based on historical data.
- Customer Behavior: Forecasting customer churn rates using past purchase data.
Prescriptive Analysis
Prescriptive analysis goes beyond predicting future outcomes by recommending actions to achieve desired results. It uses optimization and simulation techniques to provide actionable insights and decision-making support.
Key Features
- Optimization: Identifies the best course of action to achieve specific goals.
- Simulation: Models different scenarios to understand the potential impact of various decisions.
- Decision Support: Provides recommendations based on data analysis to guide decision-making.
Examples
- Supply Chain Management: Recommending optimal inventory levels to minimize costs and meet demand.
- Healthcare: Suggesting personalized treatment plans based on patient data.
Understanding the different methods of data interpretation is essential for extracting valuable insights and making informed decisions. Each method—descriptive, inferential, predictive, and prescriptive analysis—offers unique advantages and applications. By mastering these techniques, students can effectively analyze data and contribute to data-driven decision-making in various fields.
Data Visualization: The Role in Interpreting Information
Data visualization is a powerful tool for interpreting and presenting data. It involves creating visual representations of data to communicate information clearly and efficiently. By transforming complex data sets into visual formats, data visualization makes it easier to identify patterns, trends, and insights.
Importance of Visualization
- Simplifies Complex Data: Visualization helps break down complex data into understandable visuals, making it easier for audiences to grasp intricate information quickly.
- Identifies Trends and Patterns: Visualizing data enables the detection of trends and patterns that might not be obvious in raw data. This can lead to more informed decision-making.
- Enhances Data Interpretation: Visual aids like charts and graphs can highlight key findings and make data interpretation more intuitive.
- Facilitates Communication: Visual representations of data are more engaging and can communicate findings more effectively than textual descriptions alone.
- Supports Decision-Making: By providing clear and concise visuals, data visualization supports better decision-making processes in businesses and other organizations.
Types of Visualizations
Different types of visualizations serve various purposes. Choosing the right type of visualization depends on the nature of the data and the insights you want to convey.
- Charts and Graphs
- Dashboards
- Infographics
Charts and Graphs
Charts and graphs are fundamental tools in data visualization, offering a structured and visually engaging way to present data. They facilitate the understanding of data by highlighting trends, comparisons, and relationships, making it easier to draw meaningful insights.
Types of Charts and Graphs
Bar Charts
Bar charts are one of the most commonly used visualizations. They are excellent for comparing different categories or groups. Each bar’s length or height represents the value of a specific category, making it easy to see differences and trends at a glance.
- Use Case: Comparing sales figures, population sizes, or survey results across different categories.
- Example: A bar chart comparing monthly sales figures for different products. This can help identify which product performs best each month and track sales trends over time.
Line Graphs
Line graphs are ideal for showing trends over time. They use points connected by lines to represent data values, making it easy to observe changes, patterns, and trends.
- Use Case: Tracking changes over time, such as stock prices, temperature variations, or website traffic.
- Example: A line graph depicting the growth of website traffic over a year. This helps in understanding how traffic fluctuates throughout the year and identifying peak periods.
Pie Charts
Pie charts are useful for showing proportions and percentages. They represent data as slices of a pie, with each slice corresponding to a category’s contribution to the whole.
- Use Case: Displaying the market share of different companies, the distribution of budget allocations, or the composition of a population.
- Example: A pie chart illustrating market share by company. This provides a clear visual of how market share is distributed among competitors.
Scatter Plots
Scatter plots are used to show relationships between two variables. They display data points on a two-dimensional graph, with each point representing the values of two variables.
- Use Case: Identifying correlations, such as the relationship between study time and test scores, or advertising spend and sales revenue.
- Example: A scatter plot showing the relationship between advertising spend and sales. This can reveal whether increased spending correlates with higher sales, indicating the effectiveness of advertising efforts.
Histograms
Histograms are useful for displaying the distribution of data. They resemble bar charts but represent the frequency of data points within specified ranges or bins.
- Use Case: Analyzing the distribution of test scores, ages, or income levels to understand the spread and central tendency of data.
- Example: A histogram showing the distribution of customer satisfaction ratings. This can help determine how customers generally feel about a product or service and identify areas for improvement.
Charts and graphs are essential tools for data visualization, each serving different purposes depending on the nature of the data and the insights sought. By using bar charts, line graphs, pie charts, scatter plots, and histograms, students and professionals can effectively communicate data findings, making complex information more accessible and actionable. Mastering these visual tools is crucial for anyone involved in data analysis, presentation, or decision-making.
Dashboards
Dashboards are sophisticated, interactive platforms designed to consolidate multiple data visualizations into a single, easily accessible interface. They provide a comprehensive overview of key performance indicators (KPIs) and metrics, enabling users to monitor, analyze, and interpret data in real time. By presenting critical information clearly and concisely, dashboards facilitate better decision-making and performance tracking across various domains.
Features of Dashboards
Interactivity
One of the most significant features of dashboards is their interactivity. Users can engage with the data in various ways to gain deeper insights:
- Filtering: Allows users to view specific segments of data based on selected criteria, such as periods, geographic regions, or product categories.
- Drilling Down: Enables users to click on data points to see more detailed information. For example, clicking on a sales figure in a dashboard might show sales data broken down by product or region.
- Exploring Different Views: Users can switch between different visualizations and perspectives to understand data from multiple angles. This helps in identifying trends, outliers, and patterns that might not be evident from a single view.
Real-Time Updates
Dashboards can be connected to live data sources, ensuring that the information displayed is always current and accurate. This real-time data capability is crucial for time-sensitive decision-making:
- Live Data Feeds: Dashboards can pull data from various sources such as databases, APIs, and cloud services, updating automatically as new data becomes available.
- Immediate Insights: With real-time updates, users can respond quickly to emerging trends and issues. For example, a business can instantly see the impact of a marketing campaign on sales and make necessary adjustments on the fly.
Customization
Dashboards offer a high degree of customization to meet the unique needs of different users and organizations:
- Personalized Views: Users can tailor dashboards to show the most relevant metrics and KPIs for their specific roles. For instance, a sales manager might focus on revenue and lead conversion rates, while an operations manager might prioritize supply chain efficiency and inventory levels.
- Flexible Layouts: Users can arrange visualizations in a way that makes the most sense for their workflow, ensuring that critical information is easily accessible.
- Custom Metrics: Dashboards can be configured to calculate and display custom metrics that are important to the user’s goals and objectives.
Example of a Dashboard
Business Performance Dashboard
A business performance dashboard is a powerful example of how dashboards can integrate various data sources and visualizations to provide a holistic view of a company’s operations:
- Sales Figures: Visualized through bar charts or line graphs to show sales performance over different periods. This can help identify trends, peak sales times, and areas needing improvement.
- Customer Feedback: Displayed using pie charts, word clouds, or sentiment analysis graphs to gauge customer satisfaction and identify common issues or praise.
- Inventory Levels: Shown through gauges, tables, or area charts to track stock levels, turnover rates, and reorder points. This helps in managing supply chain efficiency and avoiding stockouts or overstock situations.
Dashboards are invaluable tools for modern data management and analysis. Their interactive nature, real-time updating capabilities, and a high degree of customization make them essential for anyone needing to monitor and interpret data effectively. By integrating various visualizations and data sources into a cohesive platform, dashboards empower users to make informed decisions quickly and efficiently. Mastering the use of dashboards is crucial for professionals across all industries, as it enhances their ability to leverage data for strategic advantage.
Infographics
Infographics are powerful visual tools that blend data, charts, and textual information to convey a story or message effectively. Designed to be both visually appealing and easy to understand, infographics are particularly useful for communicating complex information quickly. They leverage a combination of visual and textual elements to engage the audience and enhance comprehension, making them a popular choice for educational, marketing, and informational purposes.
Components of Infographics
Data Visualizations
Data visualizations are the backbone of infographics. They include various types of charts, graphs, and icons that represent data in a visual format. These elements help to simplify complex data sets and highlight key points in an intuitive and accessible manner.
- Charts and Graphs: Bar charts, line graphs, pie charts, and scatter plots are commonly used to display numerical data and trends.
- Icons: Icons can represent specific data points or categories, adding a visual shorthand that enhances understanding and recall.
- Maps: Geographical data can be represented using maps, which are effective for showing location-based information and trends.
Text
Text in infographics provides essential context, explanations, and insights that support the visual elements. It guides the viewer through the infographic, ensuring that the message is clear and coherent.
- Headlines and Subheadings: These draw attention to key sections and help structure the information.
- Explanatory Text: Brief explanations or annotations clarify the data and provide necessary background information.
- Data Labels: Labels on charts and graphs ensure that viewers understand what each data point represents.
Design Elements
Design elements are crucial for making infographics visually engaging and easy to navigate. Effective use of colors, fonts, and layouts can significantly enhance the readability and overall impact of an infographic.
- Colors: Strategic use of color can highlight important information, create visual harmony, and make the infographic more appealing.
- Fonts: Choosing the right fonts can improve readability and set the tone of the infographic. Different fonts can be used for headings, subheadings, and body text to create a clear visual hierarchy.
- Layouts: A well-organized layout ensures that the information flows logically and is easy to follow. Good layouts balance visual elements and text, preventing overcrowding and confusion.
Example of an Infographic
Health Statistics Infographic
A health statistics infographic is a practical example that combines charts, icons, and text to present data on health trends and statistics effectively.
- Visual Elements: The infographic might include bar charts showing the prevalence of various health conditions, line graphs depicting changes in life expectancy over time, and pie charts illustrating the distribution of healthcare spending.
- Icons: Icons representing different health conditions (e.g., heart disease, diabetes) can be used to quickly convey information about their prevalence.
- Textual Information: The text provides context for the data visualizations, such as explanations of trends, definitions of medical terms, and insights into the significance of the statistics.
- Design Elements: Colors are used to differentiate between various health conditions and statistical categories. Fonts are chosen for readability and to highlight key information, and the layout is designed to guide the viewer through the data logically.
Infographics are a highly effective way to communicate complex information in a visually engaging and easily digestible format. By combining data visualizations, text, and design elements, infographics can tell a compelling story and convey critical insights quickly. Understanding the components of a good infographic and how to use them effectively is essential for creating impactful visual content that captures and retains the audience’s attention.
Data visualization plays a crucial role in interpreting and communicating data effectively. By using various types of visualizations, such as charts, graphs, dashboards, and infographics, students can gain valuable insights and make data-driven decisions. Mastering the art of data visualization is essential for anyone looking to leverage data in their academic or professional endeavors.
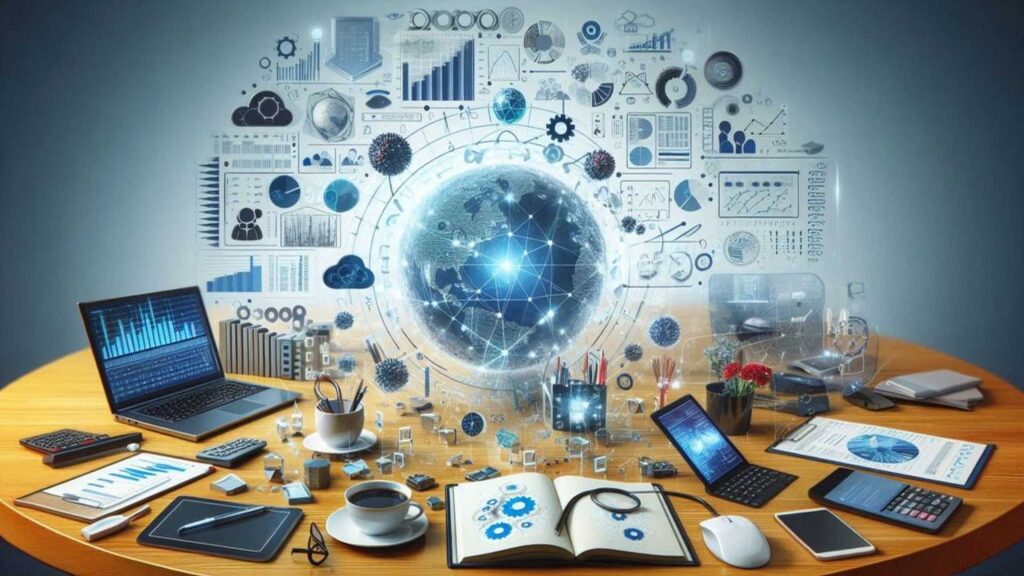
Tools for Data Interpretation
Data interpretation is a critical step in data analysis, enabling users to extract meaningful insights and make informed decisions. Several powerful tools can assist with this process, each offering unique features and capabilities. Below, we explore three essential tools for data interpretation: Excel, Tableau, and Python/R.
Excel
Excel is one of the most widely used tools for data analysis and interpretation. Its versatility and ease of use make it a favorite among students and professionals alike.
Key Features
- Data Organization: Excel allows users to organize data into rows and columns, making it easy to manage and manipulate datasets.
- Formulas and Functions: Excel offers a vast array of built-in formulas and functions for performing calculations, statistical analysis, and data manipulation.
- Pivot Tables: Pivot tables enable users to summarize large datasets quickly, providing insights into patterns and trends.
- Charts and Graphs: Excel provides various chart types, such as bar charts, line graphs, and pie charts, for visualizing data.
Example
- Sales Data Analysis: Imagine you have monthly sales data for different products. Using Excel, you can create pivot tables to summarize total sales by product, apply formulas to calculate growth rates and generate charts to visualize sales trends over time.
Tableau
Tableau is a powerful data visualization tool that enables users to create interactive and shareable dashboards. It is designed to handle large datasets and provide deep insights through visual storytelling.
Key Features
- Interactive Dashboards: Tableau’s dashboards allow users to explore data interactively, filtering and drilling down into specific details.
- Data Connectivity: Tableau can connect to various data sources, including databases, spreadsheets, and cloud services, ensuring seamless data integration.
- Advanced Visualizations: Tableau offers a wide range of visualization options, from basic charts to complex graphs and maps.
- Real-Time Data: Tableau can handle real-time data, providing up-to-date insights and enabling timely decision-making.
Example
- Customer Feedback Dashboard: Using Tableau, you can create a dashboard that visualizes customer feedback data from multiple sources. This dashboard might include charts showing customer satisfaction trends, maps highlighting regional feedback differences, and filters allowing users to explore feedback by product or period.
Python and R
Python and R are programming languages that offer robust tools for data analysis and interpretation. They are particularly popular in data science for their flexibility and extensive libraries.
Key Features
- Data Manipulation: Both Python (with libraries like Pandas) and R (with libraries like dplyr) excel at data manipulation, allowing users to clean, transform, and aggregate data efficiently.
- Statistical Analysis: Python and R provide powerful statistical analysis capabilities, including hypothesis testing, regression analysis, and more.
- Data Visualization: Libraries such as Matplotlib, Seaborn (Python), and ggplot2 (R) enable the creation of high-quality visualizations.
- Machine Learning: Python and R support machine learning and predictive modeling through libraries like Scikit-learn (Python) and Caret (R).
Example
- Predictive Modeling in Python: Suppose you want to predict future sales based on historical data. Using Python, you can import the data with Pandas, perform exploratory data analysis, and build a predictive model using Scikit-learn. Visualizations created with Matplotlib and Seaborn can help illustrate the model’s performance and predictions.
Excel, Tableau, and Python/R are invaluable tools for data interpretation, each catering to different needs and levels of complexity. Excel is ideal for quick analysis and straightforward visualizations, Tableau excels in creating interactive dashboards, and Python/R offers advanced analytical capabilities and flexibility. By mastering these tools, students can enhance their data interpretation skills and leverage data-driven insights effectively in various contexts.
Common Challenges in Data Interpretation
While data interpretation is a powerful tool, it is not without its challenges. Engineering students should be aware of these challenges to effectively navigate the data interpretation landscape and avoid potential pitfalls.
Data Quality Issues
- Incomplete or Missing Data: Datasets may have gaps or missing values, which can lead to biased or inaccurate conclusions if not properly addressed.
- Inaccurate or Erroneous Data: Data can be prone to errors, either due to human input mistakes or technical issues during data collection or processing.
- Irrelevant or Redundant Data: Datasets may contain irrelevant or redundant information that can clutter the analysis and obscure the true insights.
- Biased or Skewed Data: Data can be biased due to sampling methods, data collection procedures, or inherent biases in the population being studied.
Misinterpretation of Data
- Confusing Correlation with Causation: Just because two variables are related does not necessarily mean that one causes the other. Engineers must be cautious when making causal inferences based on correlational data.
- Overgeneralizing from Limited Data: Drawing broad conclusions from a small or unrepresentative sample can lead to erroneous generalizations that do not accurately reflect the larger population or phenomenon.
- Ignoring Confounding Variables: Failing to account for external factors that may influence the relationship between variables can lead to incomplete or misleading interpretations.
- Confirmation Bias: The tendency to interpret data in a way that confirms one’s existing beliefs or hypotheses, while ignoring or downplaying evidence that contradicts those beliefs.
- Oversimplifying Complex Relationships: Real-world phenomena are often complex, with multiple interacting variables and nonlinear relationships. Simplifying these complexities can result in oversimplified or misleading interpretations.
To overcome these challenges, students should:
- Ensure data quality by implementing robust data collection and cleaning procedures, and regularly auditing the data for completeness, accuracy, and relevance.
- Be cautious when making causal inferences, and consider alternative explanations or confounding variables that may influence the observed relationships.
- Strive for objectivity by actively seeking out contradictory evidence and challenging their assumptions and biases.
- Seek to understand the limitations and context of the data, and communicate these limitations clearly when presenting findings.
- Collaborate with cross-functional teams and seek input from subject matter experts to gain a more comprehensive understanding of the data and its implications.
By being aware of these challenges and proactively addressing them, engineering students can develop a more nuanced and effective approach to data interpretation, leading to better decision-making and problem-solving in their respective fields.
The Importance of Data Communication
Effective data communication is essential in translating complex data into actionable insights. Clear and impactful communication ensures that data-driven decisions are well-informed and broadly understood. Whether you are presenting to stakeholders, colleagues, or the public, how you convey your data can significantly affect its impact and the decisions made based on it.
Effective Communication Strategies
To communicate data effectively, consider adopting these key strategies:
- Know Your Audience
- Simplify Complex Data
- Use Storytelling Techniques
Know Your Audience
Understanding your audience is the first step in effective data communication. Different audiences have varying levels of data literacy and interest in the details of your analysis.
Tips for Knowing Your Audience
- Identify Stakeholders: Determine who will be receiving your data presentation. Are they technical experts, executives, or a general audience?
- Gauge Data Literacy: Assess the audience’s familiarity with data concepts. Tailor your presentation to their level of understanding.
- Focus on Relevance: Highlight the data points that are most relevant to your audience’s interests and needs.
Example
- Technical Audience: If presenting to data scientists, you might include detailed statistical methods and complex visualizations.
- Non-Technical Audience: For executives, focus on key insights, actionable recommendations, and high-level overviews without delving into the technical details.
Simplify Complex Data
Complex data can be overwhelming and difficult to interpret without proper simplification. Simplifying data helps make it more accessible and understandable.
Tips for Simplifying Data
- Use Clear Visuals: Employ charts, graphs, and infographics that represent your data.
- Highlight Key Points: Focus on the most critical data points and trends. Avoid overloading your audience with too much information.
- Use Comparisons: Use comparisons (e.g., year-over-year changes) to make the data more relatable and easier to grasp.
Example
- Sales Data: Instead of presenting a table with hundreds of numbers, use a bar chart to show sales growth over the last five years. Highlight the years with the most significant changes to draw attention to important trends.
Use Storytelling Techniques
Storytelling is a powerful tool in data communication. By framing data within a narrative, you can make your message more engaging and memorable.
Tips for Using Storytelling Techniques
- Create a Narrative Arc: Structure your presentation with a clear beginning, middle, and end. Start with the context, present the data, and conclude with insights and recommendations.
- Use Real-Life Examples: Incorporate case studies or anecdotes to illustrate how the data applies to real-world situations.
- Make It Personal: Relate the data to your audience’s experiences or challenges to make it more relatable and impactful.
Example
- Customer Feedback: Instead of simply showing satisfaction scores, tell a story about how a specific change in product design led to a significant increase in customer satisfaction. Use before-and-after data to support your narrative.
Effective data communication is crucial for ensuring that data insights lead to informed decision-making. By knowing your audience, simplifying complex data, and using storytelling techniques, you can make your data presentations more engaging, understandable, and impactful. Mastering these strategies will enable you to convey your data-driven insights compellingly and clearly, enhancing their overall effectiveness and reach.
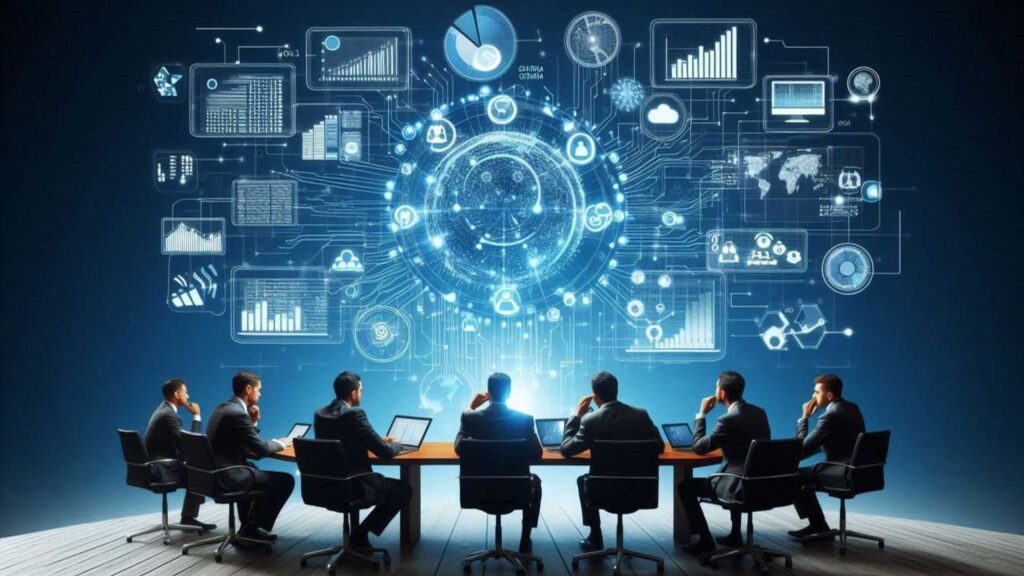
Data Interpretation and Communication: Key Best Practices
Effective data interpretation and communication are essential skills for any data analyst or researcher. By following best practices, you can ensure that your data insights are understood, actionable, and impactful. Here are some guidelines, tips, examples, and case studies to help you master these skills.
Guidelines and Tips for Effective Data Interpretation and Communication
Data interpretation and communication play a crucial role in transforming raw data into meaningful insights that drive informed decisions. Here are essential guidelines and tips to help you excel in these areas:
1. Understand the Context
Before diving into data interpretation, it’s vital to grasp the context in which the data was collected. Consider the following factors to provide accurate insights:
- Data Source: Understand where the data originates from (e.g., sales records, survey responses, IoT sensors).
- Methodology: Review how the data was collected, including sampling methods and data collection techniques.
- Limitations and Biases: Be aware of any inherent limitations or biases in the data that could impact its interpretation.
Example: When analyzing sales data, consider seasonal trends, the impact of recent marketing campaigns, and broader economic conditions that may influence sales figures. This contextual understanding provides a more nuanced interpretation of the data’s implications.
2. Focus on Key Metrics
Identify and prioritize the most critical metrics that align with your objectives or research questions. Avoid overwhelming your audience with unnecessary details that may obscure key insights.
- Objective Alignment: Choose metrics that directly relate to your goals, whether it’s improving efficiency, increasing revenue, or understanding customer behavior.
- Relevance: Focus on metrics that provide actionable insights and contribute to decision-making processes.
Example: In financial reporting, concentrate on essential metrics such as revenue growth, profitability margins, and cash flow. Instead of presenting every financial ratio, highlight those that are most pertinent to the financial health and strategic direction of the organization.
3. Use Clear and Relevant Visualizations
Visualizations are powerful tools for conveying complex data clearly and understandably. Choose visual formats that best illustrate your data and facilitate comprehension:
- Types of Visualizations: Utilize charts (e.g., bar charts, line graphs), graphs, and infographics effectively to present trends, comparisons, and distributions.
- Clarity: Ensure that visualizations are easy to interpret and accurately reflect the underlying data.
Example: Use a line graph to depict trends over time, such as monthly sales figures or website traffic. For comparing different categories of data, like market share by company, a bar chart provides a clear visual representation.
4. Ensure Data Accuracy and Integrity
Maintaining data accuracy and integrity is paramount to building trust in your findings and conclusions. Follow these steps to ensure data reliability:
- Validation: Validate data through checks and verification processes to minimize errors and inaccuracies.
- Cross-Referencing: Compare data with multiple reliable sources or previous records to confirm consistency.
- Transparency: Communicate any uncertainties, assumptions, or limitations associated with the data collection and analysis process.
Example: Before presenting survey results, validate the accuracy of data entry and ensure that survey questions were clear, unbiased, and effectively captured respondents’ perspectives. Transparently disclose any limitations, such as small sample size or demographic biases, that may affect the interpretation of findings.
5. Tell a Compelling Story
Transforming data into a compelling narrative enhances engagement and facilitates understanding among your audience. Use storytelling techniques to contextualize data and make insights memorable and actionable:
- Narrative Structure: Frame your presentation with a clear beginning, middle, and end that guides your audience through the data journey.
- Contextualization: Connect data points to real-world scenarios or challenges to illustrate their significance.
- Impact Highlighting: Emphasize how data-driven insights can lead to positive outcomes or inform strategic decisions.
Example: Instead of presenting raw data points, craft a narrative around how a customer satisfaction initiative led to increased retention rates and subsequent growth in sales. Use visuals and anecdotes to illustrate the journey and outcomes of the initiative, making the data more relatable and compelling.
By adhering to these best practices, you can enhance your proficiency in data interpretation and communication. Effective understanding of data context, focusing on key metrics, using clear visualizations, ensuring data accuracy, and telling compelling stories are essential skills that empower you to transform data into actionable insights that drive organizational success and informed decision-making. Practicing these guidelines will not only improve your data analysis capabilities but also increase the impact and relevance of your data presentations.
Examples and Case Studies
Example 1: Marketing Campaign Effectiveness
Objective: Assess the effectiveness of a recent marketing campaign aimed at increasing customer engagement.
Steps Taken:
- Analyzed campaign metrics such as click-through rates (CTR), conversion rates, and social media engagement.
- Created visualizations including line graphs for CTR trends and pie charts for channel-wise conversion rates.
- Presented findings using a dashboard that allowed stakeholders to interactively explore campaign performance.
Example 2: Academic Performance Analysis
Objective: Evaluate student performance across different subjects over multiple semesters.
Steps Taken:
- Compiled and cleaned student grade data from various courses.
- Used Excel to create pivot tables and charts showing average grades, grade distributions, and trends over time.
- Prepared a report with actionable insights for educators and administrators to improve teaching strategies.
By applying these best practices, guidelines, and examples, you can enhance your ability to interpret and communicate data effectively. Whether you’re presenting to stakeholders, colleagues, or clients, clear and insightful data communication is key to fostering understanding, making informed decisions, and driving positive outcomes. Practice these techniques to refine your skills and maximize the impact of your data-driven insights.
Applied Learning Projects for Machine Learning Beginners
Machine learning projects are invaluable for beginners, offering practical insights and hands-on experience in applying data analysis techniques to solve real-world problems. Here’s an in-depth look at three beginner-friendly projects that introduce fundamental concepts and methodologies:
1. Predictive Analytics with Linear Regression
Objective: Predict a continuous target variable based on one or more independent variables using linear regression.
Project Steps:
- Data Collection: Begin by gathering a suitable dataset. For instance, you might collect data on customer demographics, spending habits, and purchase frequency.
- Data Preprocessing: Clean and prepare the data. This involves handling missing values, encoding categorical variables, and scaling numerical features to ensure all data is in a consistent format suitable for analysis.
- Model Building: Implement a linear regression model using popular Python libraries such as scikit-learn. Train the model on a training dataset and evaluate its performance on a separate test dataset.
- Evaluation: Assess the model’s accuracy using metrics like mean squared error (MSE) or R-squared. Visualize the model’s predictions against actual values using scatter plots or regression plots to understand how well the model fits the data.
Example:
- Dataset: Use a dataset containing historical customer data, including variables like age, income, and spending habits.
- Visualization: Visualize the relationship between customer age and expenditure using a scatter plot, with the fitted linear regression line illustrating trends in spending behavior over different age groups.
2. Customer Segmentation using K-means Clustering
Objective: Group customers into distinct segments based on similarities in purchasing behavior.
Project Steps:
- Data Preparation: Obtain a dataset with customer features such as purchase frequency, average transaction value, and types of products purchased.
- Feature Scaling: Standardize numerical features to ensure all variables contribute equally to the clustering process.
- Clustering: Apply the K-means clustering algorithm using Python’s scikit-learn library. Determine the optimal number of clusters using techniques like the elbow method or silhouette score.
- Visualization: Visualize the resulting clusters using scatter plots or 3D plots, effectively illustrating how customers are grouped based on their purchasing patterns.
Example:
- Dataset: Utilize an e-commerce transaction dataset containing customer IDs, purchase frequency, and total spending.
- Visualization: Create a scatter plot that showcases clusters of customers based on variables like purchase frequency and total spending, with each cluster uniquely colored for clear differentiation.
3. Sentiment Analysis with Naive Bayes
Objective: Classify text data (e.g., customer reviews, social media comments) into positive, negative, or neutral sentiments using the Naive Bayes classifier.
Project Steps:
- Data Acquisition: Collect text data from various sources such as social media platforms or product review websites.
- Text Preprocessing: Clean and preprocess text data by removing stopwords, and punctuation, and performing tokenization to prepare it for analysis.
- Feature Extraction: Convert text data into numerical features using methods like TF-IDF (Term Frequency-Inverse Document Frequency), capturing the importance of words in documents.
- Classification: Implement the Naive Bayes classifier using libraries such as NLTK (Natural Language Toolkit) or scikit-learn. Train the model on labeled data and evaluate its performance using metrics like accuracy or F1-score.
- Visualization: Visualize the classifier’s performance using tools like confusion matrices or bar charts, effectively demonstrating its ability to correctly classify sentiments.
Example:
- Dataset: Use a Twitter dataset containing tweets labeled as positive, negative, or neutral sentiments.
- Visualization: Create a confusion matrix to showcase the classifier’s accuracy in correctly identifying sentiment categories, providing a clear representation of its performance.
These applied learning projects offer beginners practical experience in machine learning, reinforcing key concepts such as data preprocessing, model implementation, evaluation techniques, and result visualization. By completing these projects, students not only gain proficiency in using essential machine learning algorithms but also develop the skills necessary to tackle complex data analysis tasks effectively. This hands-on approach prepares learners for real-world applications and empowers them to contribute meaningfully to the field of data science.
Advanced Machine Learning Projects
Advanced machine learning projects delve deeper into complex algorithms and methodologies, providing students with comprehensive insights and practical experience. Here are three advanced projects that demonstrate sophisticated applications of machine learning techniques:
1. Image Classification with Convolutional Neural Networks (CNNs)
Objective: Develop a model to classify images into predefined categories using Convolutional Neural Networks (CNNs), known for their effectiveness in image recognition tasks.
Project Steps:
- Data Collection: Obtain a dataset containing images labeled with respective categories (e.g., animals, vehicles, flowers).
- Data Preprocessing: Resize images to a consistent format, normalize pixel values, and augment data to increase diversity and robustness.
- Model Building: Construct a CNN architecture using frameworks like TensorFlow or PyTorch. Design layers including convolutional, pooling, and fully connected layers to learn hierarchical features from images.
- Training and Evaluation: Train the CNN model on a training set, validate its performance on a validation set, and test it on a separate test set. Evaluate classification accuracy and other metrics such as precision, recall, and F1-score.
- Visualization: Visualize model predictions, confusion matrices, and feature maps to understand how CNN processes and classifies images.
Example:
- Dataset: Use the CIFAR-10 dataset with 60,000 32×32 color images across 10 classes.
- Visualization: Display sample images from different classes alongside their predicted labels to illustrate CNN’s classification accuracy and capabilities.
2. Time Series Forecasting with LSTM
Objective: Forecast future values of a time series dataset using Long Short-Term Memory (LSTM) networks, which are well-suited for sequential data analysis.
Project Steps:
- Data Collection: Gather a time series dataset (e.g., stock prices, weather data, sales figures) with historical observations.
- Data Preprocessing: Normalize data, handle missing values, and split into training and testing sets. Convert the time series data into sequences suitable for LSTM input.
- Model Building: Construct an LSTM model using libraries like TensorFlow or Keras. Configure the network with LSTM layers followed by dense layers for output prediction.
- Training and Evaluation: Train the LSTM model on the training set, validate performance on a validation set, and evaluate accuracy using metrics like Mean Absolute Error (MAE) or Root Mean Square Error (RMSE).
- Visualization: Plot actual vs. predicted values over time to visualize the model’s forecasting accuracy and trends.
Example:
- Dataset: Use a historical stock price dataset, predicting future stock prices based on past trends and market conditions.
- Visualization: Display a line graph showing actual stock prices alongside LSTM predictions, highlighting the model’s ability to capture trends and fluctuations.
3. Reinforcement Learning for Game Playing
Objective: Develop an agent capable of learning optimal strategies for playing games using Reinforcement Learning (RL), a branch of machine learning focused on decision-making and behavior learning.
Project Steps:
- Environment Setup: Choose a game environment (e.g., Tic-Tac-Toe, Atari games) suitable for RL experimentation.
- State Representation: Define how game states are represented as input to the RL agent (e.g., board configurations, pixel frames).
- Model Building: Implement RL algorithms such as Q-Learning or Deep Q-Networks (DQN) using frameworks like OpenAI Gym or TensorFlow Agents. Design neural networks or Q-tables to learn and update policies based on rewards and penalties.
- Training and Evaluation: Train the RL agent through interactions with the game environment, adjusting policies to maximize rewards. Evaluate performance by measuring win rates, scores, or other game-specific metrics.
- Visualization: Visualize the RL agent’s gameplay sessions, showing strategies learned over time and comparing performance before and after training.
Example:
- Game: Use the classic Atari game “Pong” where the RL agent learns to control paddles to win against opponents.
- Visualization: Showcase gameplay videos or animations demonstrating the RL agent’s progression from random actions to learned strategies for winning matches.
These advanced machine-learning projects provide students with deep insights into complex algorithms and methodologies, preparing them for challenging real-world applications. By engaging in these projects, learners gain hands-on experience in building and deploying advanced machine learning models, honing their skills in data preprocessing, model training, evaluation, and result visualization. This practical approach not only enhances technical proficiency but also fosters creativity and problem-solving abilities essential for succeeding in the dynamic field of machine learning.
Data Science Projects
Data science projects offer practical applications of analytical techniques to derive insights and solutions from data. Here are three engaging projects that provide valuable learning experiences:
1. Exploratory Data Analysis (EDA) on Retail Data
Objective: Perform Exploratory Data Analysis (EDA) on a retail dataset to uncover patterns, trends, and relationships that can inform business decisions.
Project Steps:
- Data Acquisition: Obtain a retail dataset containing information such as sales transactions, customer demographics, and product details.
- Data Cleaning: Clean and preprocess the data by handling missing values, removing duplicates, and standardizing formats.
- Exploratory Analysis: Conduct descriptive statistics, visualizations (e.g., histograms, box plots, scatter plots), and correlation analysis to understand the dataset’s characteristics.
- Insights Generation: Identify key insights such as popular products, seasonal trends in sales, customer segmentation based on purchasing behavior, and correlations between variables.
- Visualization Example: Create a dashboard or series of visualizations showcasing sales trends over time, customer demographics, and product performance metrics.
2. Building Recommendation Systems
Objective: Develop a recommendation system that suggests items (e.g., products, movies) to users based on their preferences and historical interactions.
Project Steps:
- Data Collection: Gather a dataset with user-item interactions (e.g., ratings, purchase history) suitable for building a recommendation engine.
- Data Preprocessing: Transform data into a suitable format (e.g., user-item matrices) and handle sparse or missing values.
- Model Selection: Choose a recommendation algorithm such as collaborative filtering (user-based or item-based), content-based filtering, or hybrid methods.
- Model Training: Train the recommendation model on historical data to learn user preferences and item similarities.
- Evaluation: Assess the model’s performance using metrics like precision, recall, and Mean Average Precision (MAP). Use techniques like cross-validation to validate results.
- Visualization Example: Display personalized recommendations for users through a user-friendly interface or visualization tool.
3. Fraud Detection using Machine Learning
Objective: Develop a machine learning model to detect fraudulent transactions or activities in financial or e-commerce datasets.
Project Steps:
- Data Collection: Obtain a dataset containing transactions with labeled fraud indicators (fraudulent or non-fraudulent).
- Data Preprocessing: Clean and preprocess data, handling imbalanced classes, scaling numerical features, and encoding categorical variables.
- Model Building: Choose suitable algorithms such as Logistic Regression, Random Forest, or Gradient Boosting. Train the model to distinguish between fraudulent and non-fraudulent transactions.
- Evaluation: Evaluate model performance using metrics like accuracy, precision, recall, and Area Under the Receiver Operating Characteristic Curve (AUC-ROC).
- Visualization Example: Create visualizations such as confusion matrices or ROC curves to illustrate the model’s ability to detect fraud effectively.
These data science projects offer students valuable opportunities to apply theoretical knowledge in practical settings. By engaging in projects like exploratory data analysis, building recommendation systems, and fraud detection, learners gain hands-on experience in data preprocessing, model selection, evaluation, and result interpretation. These skills are essential for aspiring data scientists to address real-world challenges and contribute effectively to data-driven decision-making in various industries.
Real-World Applications of Data Science
Data science finds extensive applications across various sectors, influencing decision-making processes and fostering innovation. Here’s an exploration of its impact on business, healthcare, and education:
1. Business Decision-Making
Role of Data Science: In business, data science plays a pivotal role in leveraging data to gain insights that drive strategic decisions and enhance operational efficiency.
Applications:
- Market Analysis: Analyzing customer preferences and market trends through data enables businesses to tailor products and services to meet consumer demands effectively.
- Predictive Analytics: Forecasting sales, demand trends, and customer behavior using machine learning models helps businesses optimize inventory management and marketing strategies.
- Customer Segmentation: Segmenting customers based on purchasing patterns and demographics allows businesses to personalize marketing campaigns and improve customer retention.
- Risk Management: Using data science techniques for risk assessment and fraud detection helps mitigate financial risks and maintain business integrity.
Example:
- Visualization: A dashboard displaying real-time sales data, customer demographics, and market performance metrics helps business leaders make informed decisions on product launches and marketing investments.
2. Healthcare
Role of Data Science: Data science transforms healthcare by enabling evidence-based decision-making, improving patient outcomes, and optimizing healthcare delivery.
Applications:
- Medical Diagnostics: Analyzing medical imaging data (e.g., X-rays, MRI scans) using machine learning algorithms aids in early disease detection and accurate diagnosis.
- Personalized Medicine: Using patient data (genomic information, medical history) to tailor treatment plans and predict treatment response enhances medical interventions.
- Healthcare Operations: Optimizing hospital resource allocation, patient scheduling, and healthcare logistics improves efficiency and reduces costs.
- Public Health Surveillance: Monitoring disease outbreaks, predicting healthcare trends, and allocating resources based on predictive models enhances public health initiatives.
Example:
- Visualization: A heat map illustrating disease prevalence across different regions helps public health officials allocate resources for targeted intervention and prevention strategies.
3. Education
Role of Data Science: In education, data science empowers educators and administrators to enhance learning outcomes, personalize education experiences, and optimize educational processes.
Applications:
- Learning Analytics: Analyzing student performance data (e.g., test scores, learning activities) helps educators identify at-risk students, personalize learning paths, and provide timely interventions.
- Predictive Modeling: Forecasting student outcomes and identifying factors influencing academic success supports proactive educational strategies and student support services.
- Curriculum Optimization: Using data-driven insights to revise curriculum, update teaching methodologies, and improve educational resources enhances learning effectiveness.
- Administrative Efficiency: Optimizing administrative processes such as student enrollment, resource allocation, and educational planning streamlines operations and improves institutional performance.
Example:
- Visualization: A dashboard displaying student progress, engagement metrics, and performance trends enables educators to track individual student growth and identify areas needing improvement.
These real-world applications highlight the transformative impact of data science across diverse sectors. By harnessing the power of data analytics, businesses optimize decision-making, healthcare providers enhance patient care, and educators personalize learning experiences. Understanding these applications not only prepares students for careers in data science but also underscores the importance of data-driven approaches in driving innovation and addressing societal challenges effectively.
Future Trends in Data Interpretation and Communication
As data interpretation and communication continue to evolve, several key trends are shaping the landscape of data science and engineering. These trends highlight advancements in AI and machine learning, automation in data analytics, and the growing importance of ethical considerations in data science practices.
AI and Machine Learning in Data Interpretation
AI and machine learning are revolutionizing the field of data interpretation by enabling more efficient and accurate analysis of large, complex datasets. Some key trends and applications include:
- Natural Language Processing (NLP): Allows computers to understand, interpret, and respond to human language, enabling analysis of unstructured text data like customer reviews and social media posts.
- Predictive Analytics: Uses machine learning algorithms to forecast future trends and outcomes based on historical data, helping organizations make more informed decisions.
- Augmented Analytics: Automates data preparation and presentation using machine learning, producing rapid insights in data-driven domains.
- Cognitive Computing: Refers to AI systems that engage with customers through natural language interactions, capturing real-time data and insights.
Automation and Data Analytics
Automation is a critical enabler of advanced data analytics, allowing for the processing of vast amounts of data at scale. Some key trends include:
- Internet of Things (IoT): The growth of IoT is driving increased data generation and the need for advanced analytics to extract value from this data.
- Edge Computing: Enables data processing closer to the source, solving connectivity and latency challenges associated with data travel in IoT environments.
- Cloud Services: Offer scalable and cost-effective storage and processing power for big data analytics.
- Hybrid Data Sources: Combining cloud and on-premises data sources can provide a more comprehensive view for analysis.
Ethical Considerations in Data Science
As data science and analytics become more advanced and pervasive, it is crucial to consider the ethical implications and ensure the responsible development and use of these technologies. Key considerations include:
- Data Privacy and Security: Protecting sensitive data and ensuring compliance with regulations like GDPR.
- Algorithmic Bias: Identifying and mitigating biases in machine learning models that can lead to unfair or discriminatory outcomes.
- Transparency and Explainability: Making AI systems more interpretable and understandable, especially for high-stakes decisions.
- Human-AI Collaboration: Ensuring that AI augments and empowers human decision-making rather than replacing it entirely.
- Environmental and Social Impact: Considering the broader societal and environmental implications of data-driven technologies and their applications.
By embracing these trends while prioritizing ethical considerations, engineering students can harness the power of data interpretation and communication to drive innovation, solve complex problems, and create a positive impact in their fields.
Conclusion
As we conclude this on data interpretation and communication for students, it’s important to emphasize the significance of these skills in today’s data-driven world. The ability to effectively interpret and communicate insights from data is no longer a luxury, but a necessity for success in any engineering field.
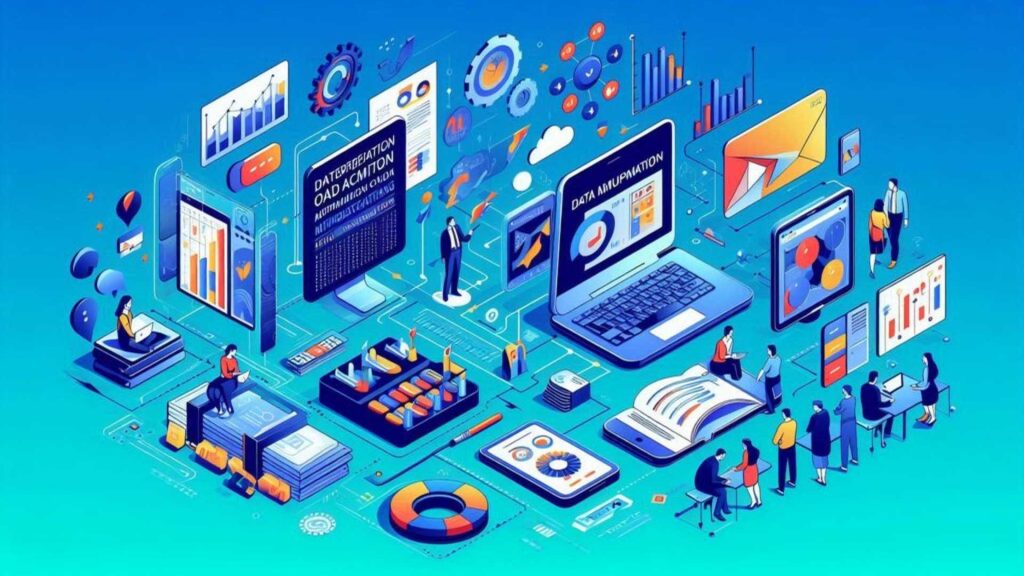
Recap of Key Points
In this article, we have explored the importance of data interpretation and communication for engineering students. We discussed the following key points:
- Importance of Data Interpretation: Effective data interpretation is crucial for informed decision-making, identifying trends and patterns, communicating insights, and driving continuous improvement.
- Key Concepts and Terminology: We covered essential concepts such as data collection, data cleaning, descriptive statistics, exploratory data analysis, inferential statistics, data visualization, and storytelling with data.
- Common Challenges in Data Interpretation: We highlighted challenges like data quality issues, misinterpretation of data, confusing correlation with causation, and the importance of addressing these challenges.
- Future Trends: We explored the impact of AI and machine learning, the role of automation and data analytics, and the ethical considerations in data science, emphasizing the need for responsible development and use of these technologies.
Final Thoughts
As the world becomes increasingly data-driven, the ability to effectively interpret and communicate data-driven insights is a critical skill for engineering students. By mastering the concepts and techniques covered in this article, you can position yourself as a valuable asset in your field, capable of making informed decisions, solving complex problems, and driving innovation.
Remember, data interpretation is not just about numbers and statistics – it’s about uncovering meaningful insights, telling compelling stories, and using data to create positive change. Embrace the challenges, stay curious, and continuously expand your knowledge and skills in this dynamic and ever-evolving field.
Embark on your data interpretation journey with enthusiasm, rigor, and a commitment to ethical practices. The insights and solutions you uncover have the power to transform industries, improve lives, and shape the future.
To seize this opportunity, we need a program that empowers the current IT student community with essential fundamentals in data science, providing them with industry-ready skills aligned with their academic pursuits at an affordable cost. Trizula Mastery in Data Science is the perfect fit for aspiring professionals, equipping them with the necessary fundamentals in contemporary technologies such as data science, and laying the groundwork for advanced fields like AI, ML, NLP, Data Visualization, and deep science. Our program is committed to equipping engineering students with marketable skills that will serve as a solid foundation for future professional advancement. Click here to get started!
FAQ’s :
1. What is communication in data science?
Communication in data science involves effectively conveying insights, findings, and recommendations derived from data analysis to stakeholders, colleagues, and decision-makers. It requires translating complex technical concepts into clear, concise, and actionable information that non-technical audiences can understand and utilize.
2. What is interpretation in data science?
Interpretation in data science is the process of extracting meaningful insights and patterns from data. It involves applying statistical and analytical techniques to raw data, identifying trends, relationships, and anomalies, and drawing conclusions that can inform decision-making and drive business value.
3. What are the four types of data interpretation?
The four types of data interpretation are:
- Descriptive interpretation: Summarizing the key characteristics of a dataset, such as measures of central tendency and dispersion.
- Exploratory interpretation: Investigating and visualizing data to uncover patterns, trends, and relationships.
- Inferential interpretation: Drawing conclusions about a population based on sample data, using techniques like hypothesis testing and regression analysis.
- Predictive interpretation: Using data to forecast future trends and outcomes based on historical patterns.
4. What are the steps of data interpretation?
The steps of data interpretation are:
- Data collection: Gathering relevant data from various sources.
- Data cleaning and preprocessing: Addressing missing values, outliers, and inconsistencies to ensure data quality and reliability.
- Exploratory data analysis: Investigating and visualizing data to uncover patterns, trends, and relationships.
- Hypothesis formulation: Developing testable hypotheses based on the initial data exploration.
- Statistical analysis: Applying appropriate statistical techniques to test hypotheses and draw conclusions.
- Interpretation and communication: Translating the results of the analysis into actionable insights and communicating them effectively to stakeholders.
5. What are the 5 basic components of data communication?
The five basic components of data communication are:
- Message: The data being transmitted, which can include numbers, text, images, audio, or video.
- Sender: The device or system that initiates the data transmission.
- Receiver: The device or system that receives the transmitted data.
- Medium: The physical or wireless channel through which the data is transmitted, such as cables or radio waves.
- Protocol: The set of rules and standards that govern the communication process, ensuring that the sender and receiver can understand each other.