Data Science Skills: Insights, Analysis, and Innovation
In today’s digital era, data science skills are pivotal for innovation and progress. From dissecting consumer behavior to forecasting market trends, the field plays a crucial role in shaping diverse industries. Data science encompasses a range of techniques and tools for analyzing and interpreting data and driving decision-making processes. Its significance in modern industry is profound, offering a multitude of career opportunities for those proficient in its methodologies and applications.
What is Data Science?
Data science is an interdisciplinary field that employs scientific methods, algorithms, and systems to extract insights and knowledge from structured and unstructured data. It encompasses various techniques such as data mining, machine learning, and statistical analysis to uncover patterns, trends, and correlations.
The Scope of Data Science
The scope of data science extends across diverse domains including business, healthcare, finance, and technology. It involves collecting, processing, and analyzing vast amounts of data to derive actionable insights that drive strategic decision-making and innovation.
Importance of Data Science in Modern Industry
- Driving Informed Decision Making: Data science empowers organizations to make data-driven decisions by providing valuable insights into consumer preferences, market dynamics, and operational efficiencies.
- By leveraging data analytics, businesses can optimize processes, mitigate risks, and capitalize on emerging opportunities.
- Enhancing Operational Efficiency: In today’s competitive landscape, efficiency is key to success.
- Data science enables companies to streamline operations, automate repetitive tasks, and improve resource allocation.
- By harnessing the power of data, organizations can optimize workflows and enhance productivity across departments.
- Facilitating Innovation: Data science fuels innovation by uncovering hidden patterns and trends that spark new ideas and solutions.
- From developing personalized products to enhancing customer experiences, data-driven innovation drives continuous improvement and market differentiation.
- Meeting Customer Needs: Understanding customer preferences and behaviors is essential for business growth.
- Data science enables companies to gather, analyze, and interpret customer data to deliver personalized products and services.
- By catering to individual needs and preferences, organizations can foster customer loyalty and drive revenue growth.
Overview of Data Science Career Opportunities
- Data Scientist: Data scientists are responsible for collecting, analyzing, and interpreting complex data sets to inform business decisions and strategies.
- They possess a blend of technical skills, domain expertise, and analytical prowess to extract valuable insights from data.
- Data Analyst: Data analysts specialize in collecting, processing, and visualizing data to identify trends, patterns, and correlations.
- They play a crucial role in helping organizations make data-driven decisions and optimize business processes.
- Machine Learning Engineer: Machine learning engineers design, develop, and deploy machine learning models and algorithms to automate tasks and improve decision-making processes.
- They possess expertise in programming languages, algorithms, and statistical modeling techniques.
- Business Intelligence Analyst: Business intelligence analysts leverage data analytics tools and techniques to provide actionable insights and recommendations to stakeholders.
- They play a vital role in transforming raw data into meaningful business insights that drive strategic decision-making.
The Indian Engineering Education System
Data Science Programs and Indian Engineering Curriculum
- Undergraduate Programs
- Bachelor of Technology (B.Tech) and Bachelor of Engineering (B.E)
- The B.Tech and B.E degrees are the most common undergraduate programs for engineering in India.
- These programs span four years, divided into eight semesters.
- The first year typically covers foundational subjects, providing a broad base of knowledge in sciences and basic engineering principles.
- From the second year onwards, students choose their specializations, such as Computer Science, Electrical Engineering, Mechanical Engineering, Civil Engineering, etc.
- The curriculum includes theoretical classes, laboratory work, and project-based learning.
- Bachelor of Technology (B.Tech) and Bachelor of Engineering (B.E)
- Postgraduate Programs
- Master of Technology (M.Tech) and Master of Engineering (M.E)
- These postgraduate programs are designed for students seeking advanced knowledge and specialization.
- The duration is generally two years, divided into four semesters.
- The curriculum is research-oriented, with a focus on advanced technical courses and a significant research project or thesis.
- Students can specialize further in sub-disciplines of their undergraduate major or shift to related interdisciplinary fields.
- Master of Technology (M.Tech) and Master of Engineering (M.E)
- Doctoral Programs
- Ph.D. in Engineering
- Doctoral programs aim at developing research capabilities and contributing original knowledge to the field.
- The duration varies from three to five years, depending on the research topic and progress.
- Ph.D. candidates undertake coursework in the initial phase, followed by comprehensive exams and focused research.
- The program culminates in a dissertation that must be defended before a panel of experts.
- Ph.D. in Engineering
- Accreditation and Regulation
- All India Council for Technical Education (AICTE)
- The AICTE is the statutory body responsible for coordinating, planning, and managing the technical education system in India.
- It sets the standards for course curricula, faculty qualifications, and infrastructure requirements.
- National Board of Accreditation (NBA)
- The NBA assesses and accredits engineering programs to ensure quality and relevance in the education provided.
- Accreditation by the NBA is a mark of assurance that the educational institution meets the required standards.
- All India Council for Technical Education (AICTE)
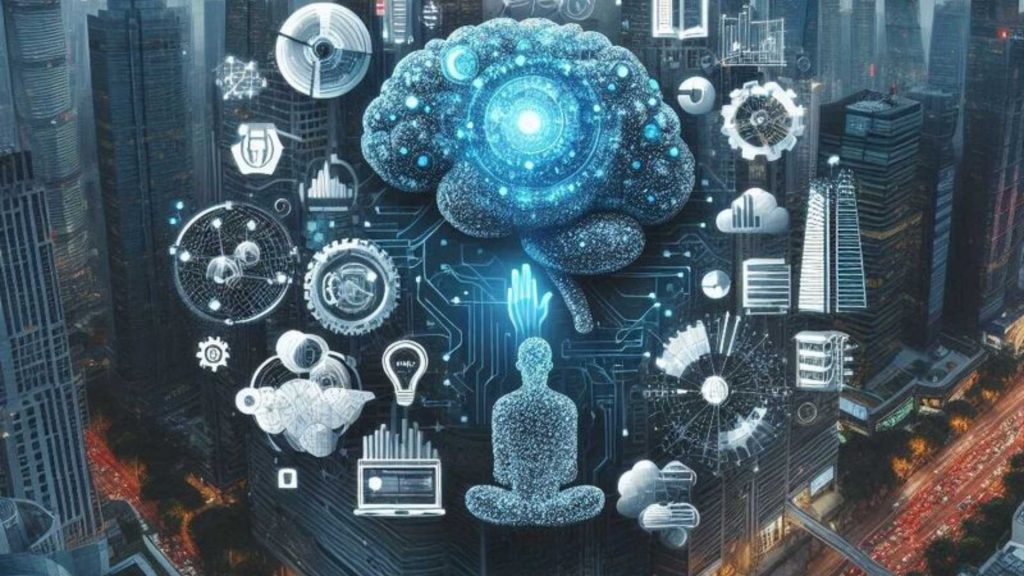
Emphasis on Technical and Analytical Skills
- Core Technical Subjects
- The curriculum starts with fundamental subjects such as Mathematics, Physics, and Chemistry.
- Specialized subjects include disciplines like Electrical Engineering, Mechanical Engineering, Civil Engineering, Computer Science, etc.
- Courses are designed to build a solid technical foundation and progressively cover advanced topics.
- Laboratory and Practical Work
- Practical sessions in laboratories are integral to the engineering curriculum.
- These sessions allow students to apply theoretical knowledge to real-world scenarios, enhancing their understanding and skills.
- Projects and assignments often require collaborative work, simulating industry conditions.
- Analytical Skill Development
- Courses are designed to foster problem-solving and critical-thinking abilities.
- Engineering Mathematics is a key component, providing tools for modeling and solving complex problems.
- Computational tools and software training (such as MATLAB, AutoCAD, etc.) are incorporated to enhance analytical capabilities.
- Internships and Industrial Training
- Industrial internships are typically mandatory, offering students exposure to practical applications of their studies.
- These internships bridge the gap between academic learning and industry requirements, providing hands-on experience.
- Workshops and seminars by industry professionals are regularly organized to keep students updated on current trends and practices.
Data Science full course integrated in Engineering Curricula
- Introduction to Data Science
- Basic courses introduce students to the core concepts of data science, including data collection, processing, and analysis.
- Foundational courses in statistics, probability, and data mining equip students with essential skills for handling data.
- These introductory courses are often offered in the second or third year of the undergraduate program.
- Specialized Data Science Courses
- Advanced courses cover machine learning, artificial intelligence, and big data analytics.
- Students learn programming languages like Python and R, which are essential for data science applications.
- Courses are designed to include practical exercises and projects to reinforce theoretical learning.
- Interdisciplinary Approach
- Data science principles are integrated into traditional engineering disciplines to enhance their scope and applications.
- For example, mechanical engineering students might use predictive maintenance techniques, while civil engineering students might work on smart infrastructure projects.
- Electrical engineering can benefit from smart grid technologies and optimization through data analytics.
- Capstone Projects and Research
- Final-year projects often involve data-driven research, allowing students to apply their data science knowledge.
- Capstone projects encourage innovation and practical application of interdisciplinary knowledge.
- Students are encouraged to publish their research findings in journals and present them at conferences.
- Collaborations and Industry Partnerships
- Engineering institutions often partner with tech companies and research institutions to stay at the forefront of technological advancements.
- Guest lectures, workshops, and collaborative research initiatives provide students with insights into industry practices and emerging trends.
- These collaborations often lead to internships and job placements, providing a smooth transition from academia to industry.
Motivation for Learning Data Science
- Intellectual Curiosity and Problem-Solving:
- Data science appeals to those with a passion for uncovering hidden patterns and solving complex problems.
- The field offers a unique blend of mathematics, statistics, and computer science, providing a rich environment for intellectually stimulating work.
- Versatile Skill Set:
- Skills in data science are applicable across a wide range of industries, from healthcare to finance, marketing, and technology.
- Learning data science opens doors to diverse job roles like data analyst, data engineer, machine learning engineer, and business analyst.
- Impactful Work:
- Data scientists often work on projects that have significant impacts on business strategies and decisions.
- The ability to drive innovation and efficiency in processes through data insights is a powerful motivator.
Career Prospects and Job Market Demand
- Growing Demand:
- The demand for data scientists is outpacing supply, leading to numerous job opportunities.
- Many industries are undergoing digital transformation, increasing the need for data-driven decision-making.
- Diverse Opportunities:
- Data science roles are available in various sectors, including technology, healthcare, finance, retail, and government.
- The versatility of data science skills means professionals can transition across different domains with relative ease.
- Global Opportunities:
- The global nature of data science allows professionals to find opportunities in numerous countries, often with options for remote work.
- Tech hubs like Silicon Valley, London, and Bangalore are major centers for data science jobs.
Salary and Job Satisfaction in Data Science Roles
- Competitive Salaries:
- Data scientists typically earn higher-than-average salaries due to the specialized skills required and high demand.
- According to recent surveys, the average salary for a data scientist can range from $95,000 to $130,000 in the US, with higher figures in top tech companies and leadership roles.
- Job Satisfaction:
- High job satisfaction is reported among data scientists due to factors like challenging work, professional growth opportunities, and the impact of their work.
- The ability to work on cutting-edge technologies and innovative projects contributes significantly to job satisfaction.
- Career Progression:
- Data science offers clear career progression paths, from junior data scientist to senior data scientist, and eventually to roles like data science manager or chief data officer.
- Continuous learning and development are integral parts of the career, with many professionals pursuing further education and certifications.
Influence of Technological Advancements and Industry Trends
- Advancements in Machine Learning and AI:
- Rapid advancements in machine learning and artificial intelligence are expanding the capabilities and applications of data science.
- Techniques like deep learning and natural language processing are opening new frontiers in areas such as image recognition and sentiment analysis.
- Big Data and Cloud Computing:
- The rise of big data technologies and cloud computing platforms like AWS, Google Cloud, and Azure is revolutionizing how data is stored, processed, and analyzed.
- These technologies enable data scientists to handle vast amounts of data more efficiently and deploy scalable solutions.
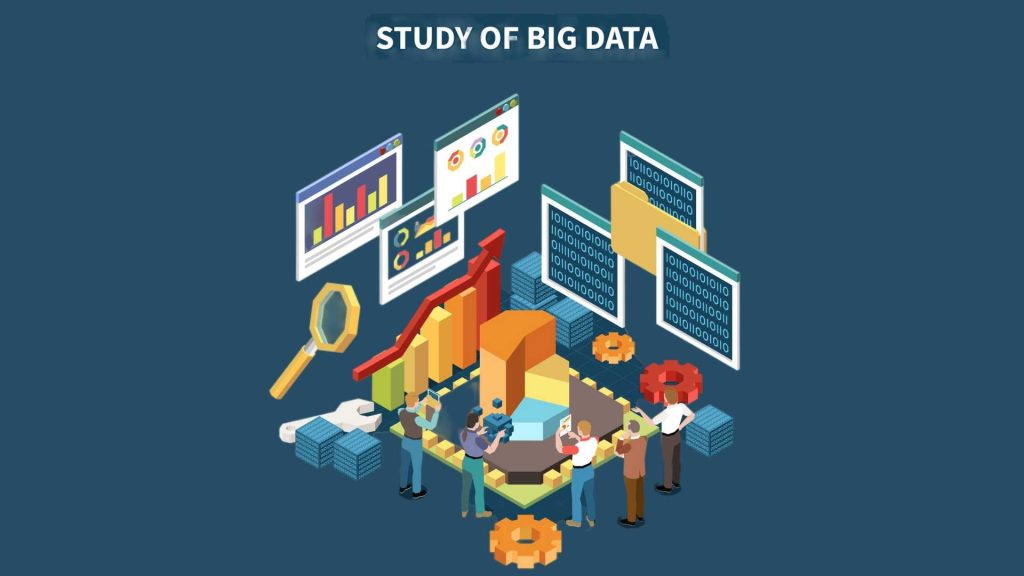
- Automation and Tools:
- Automation tools and platforms are streamlining data science workflows, making it easier to perform complex analyses and deploy machine learning models.
- Tools like TensorFlow, PyTorch, and Jupyter Notebooks are standardizing and simplifying the data science process.
- Ethics and Privacy:
- With increasing awareness of data privacy and ethical considerations, data scientists are now focusing on responsible AI and ethical data use.
- Regulatory frameworks like GDPR are shaping how data is collected, stored, and utilized, ensuring transparency and accountability.
Key Data Science Skills
Programming Languages: Python, R, and SQL
Python
- Versatility: Python is widely used due to its simplicity and readability, making it ideal for beginners and experts alike.
- Libraries and Frameworks: Extensive libraries such as NumPy, pandas, and sci-kit-learn facilitate data manipulation, analysis, and machine learning.
- Community Support: Strong community support with extensive documentation and tutorials available online.
- Integration: Seamlessly integrates with other languages and technologies, enhancing its utility in various data science workflows.
R
- Statistical Analysis: R excels in statistical analysis and is preferred for academic and research purposes.
- Data Visualization: Libraries like ggplot2 and Shiny enable advanced data visualization and interactive web applications.
- Comprehensive Packages: CRAN (Comprehensive R Archive Network) hosts thousands of packages for various data science needs.
- Built-in Support: Native support for data handling and statistical modeling functions.
SQL
- Database Management: Essential for querying and managing relational databases.
- Data Manipulation: Efficiently handle large datasets and perform complex queries with SQL commands.
- Integration: Works well with other data tools and programming languages.
- Performance: Optimizes data retrieval and storage, crucial for big data applications.
Machine Learning and Artificial Intelligence
Supervised Learning
Algorithms
- Linear Regression:
- Description: A linear approach to modeling the relationship between a dependent variable and one or more independent variables.
- Applications: Used in predictive modeling, trend forecasting, and risk assessment.
- Advantages: Simple to implement and interpret, works well with linearly separable data.
- Logistic Regression:
- Description: A regression analysis is used for binary classification problems.
- Applications: Commonly used in credit scoring, marketing campaigns, and medical diagnosis.
- Advantages: Provides probabilities and odds, interpretable coefficients.
- Decision Trees:
- Description: A non-parametric model that splits data into subsets based on feature values, forming a tree-like structure.
- Applications: Widely used in finance, healthcare, and customer segmentation.
- Advantages: Easy to visualize and interpret and handles numerical and categorical data.
- Support Vector Machines (SVM):
- Description: A supervised learning model that finds the optimal hyperplane that maximizes the margin between classes.
- Applications: Effective in high-dimensional spaces, such as text classification and image recognition.
- Advantages: Robust to overfitting in high-dimensional spaces, effective with a clear margin of separation.
- k-Nearest Neighbors (k-NN):
- Description: A lazy learning algorithm classifies data points based on the majority class among its k-nearest neighbors.
- Applications: Pattern recognition, data imputation, and recommendation systems.
- Advantages: Simple to implement, makes no assumptions about data distribution.
Model Evaluation
- Accuracy: The proportion of true results (both true positives and true negatives) among the total number of cases examined.
- Precision: The ratio of correctly predicted positive observations to the total predicted positives.
- Recall: The ratio of correctly predicted positive observations to all observations in the actual class.
- F1-Score: The harmonic mean of precision and recall, balancing the two.
- ROC-AUC: The area under the receiver operating characteristic curve, measuring the ability of the model to distinguish between classes.
Cross-Validation
- K-Fold Cross-Validation: The dataset is divided into k subsets, and the model is trained k times, each time using a different subset as the validation set and the remaining k-1 subsets as the training set.
- Stratified K-Fold Cross-Validation: Ensures each fold has the same proportion of classes as the original dataset, useful for imbalanced datasets.
- Leave-One-Out Cross-Validation (LOOCV): A special case of k-fold where k equals the number of data points, used for small datasets.
Unsupervised Learning
Clustering
- K-Means:
- Description: Partitions data into k clusters by minimizing the variance within each cluster.
- Applications: Market segmentation, image compression, and document clustering.
- Advantages: Simple to implement, scalable to large datasets.
- Hierarchical Clustering:
- Description: Builds a hierarchy of clusters using either a bottom-up (agglomerative) or top-down (divisive) approach.
- Applications: Gene expression analysis, social network analysis.
- Advantages: No need to specify the number of clusters beforehand, produces a dendrogram for visualization.
- DBSCAN (Density-Based Spatial Clustering of Applications with Noise):
- Description: Groups together points that are closely packed together while marking points in low-density regions as outliers.
- Applications: Geographic data analysis, and anomaly detection in network traffic.
- Advantages: Identifies arbitrarily shaped clusters, robust to noise.
Dimensionality Reduction
- PCA (Principal Component Analysis):
- Description: Reduces the dimensionality of data by transforming to a new set of variables (principal components) that retain the most variance.
- Applications: Data visualization, noise reduction, feature extraction.
- Advantages: Simplifies data while retaining essential trends and patterns.
- t-SNE (t-Distributed Stochastic Neighbor Embedding):
- Description: A non-linear dimensionality reduction technique that models high-dimensional data for visualization in a low-dimensional space.
- Applications: Visualizing clusters, and understanding the structure of high-dimensional data.
- Advantages: Effective for visualizing high-dimensional data in 2 or 3 dimensions.
- LDA (Linear Discriminant Analysis):
- Description: Finds a linear combination of features that best separates two or more classes of objects or events.
- Applications: Pattern recognition, face recognition.
- Advantages: Enhances class separability, useful for dimensionality reduction in supervised settings.
Anomaly Detection
- Description: Identifying rare items, events, or observations that raise suspicions by differing significantly from the majority of the data.
- Applications: Fraud detection, network security, fault detection in machinery.
- Techniques: Statistical methods, proximity-based methods (e.g., k-NN), clustering-based methods (e.g., DBSCAN).
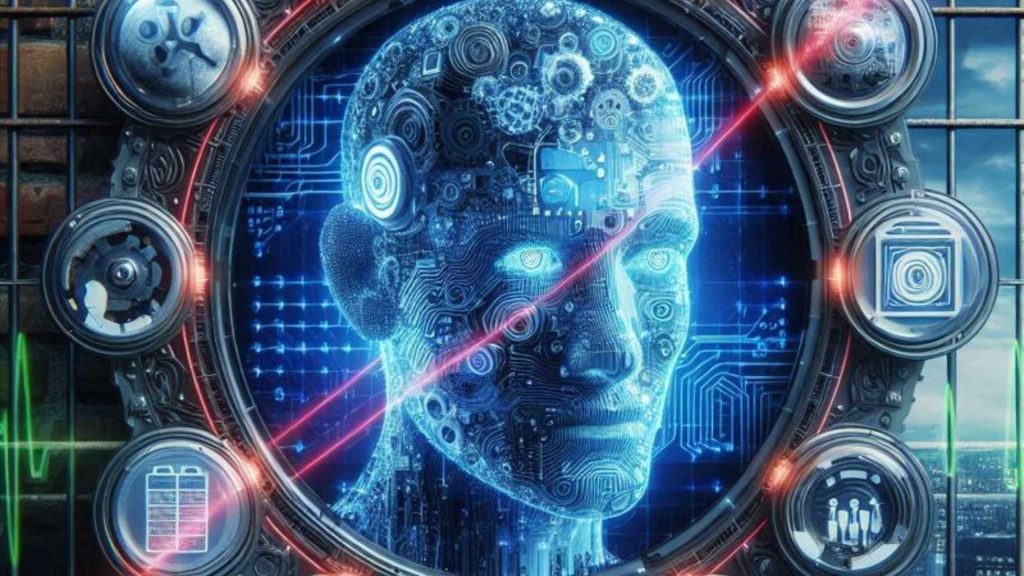
Deep Learning
Neural Networks
- Basics: Composed of layers of interconnected nodes (neurons), where each connection has a weight-adjusted during training.
- Activation Functions: Functions like ReLU (Rectified Linear Unit), Sigmoid, and Tanh introduce non-linearity into the network.
- Backpropagation: The algorithm used for training neural networks, involves forward propagation to calculate output and backward propagation to update weights.
Architectures
- Convolutional Neural Networks (CNNs):
- Description: Specialized neural networks for processing structured grid data like images.
- Components: Convolutional layers, pooling layers, fully connected layers.
- Applications: Image and video recognition, medical image analysis.
- Recurrent Neural Networks (RNNs):
- Description: Networks with loops that allow information to persist, ideal for sequential data.
- Variants: LSTM (Long Short-Term Memory) and GRU (Gated Recurrent Unit) for improved memory retention.
- Applications: Language modeling, time series prediction, speech recognition.
Frameworks
- TensorFlow: An open-source framework developed by Google, widely used for building and deploying machine learning models.
- Keras: A high-level neural networks API, running on top of TensorFlow, simplifying the creation of deep learning models.
- PyTorch: An open-source deep learning framework developed by Facebook’s AI Research lab, known for its dynamic computation graph and ease of use.
Reinforcement Learning
Key Concepts
- Agents: Entities that perform actions in an environment to achieve a goal.
- Environments: The world or system in which the agent operates.
- States: Representations of the current situation of the agent within the environment.
- Actions: Choices made by the agent that affect the environment.
- Rewards: Feedback from the environment based on the agent’s actions, guiding the learning process.
Algorithms
- Q-Learning: A model-free reinforcement learning algorithm that learns the value of actions in states through trial and error.
- Deep Q-Networks (DQN): Combines Q-learning with deep neural networks to handle high-dimensional state spaces.
- Policy Gradients: Directly optimizes the policy by computing the gradient of expected reward for the policy parameters.
Data Visualization and Communication
Data Visualization Tools
Matplotlib and Seaborn
- Matplotlib: A foundational plotting library in Python, used for creating static, animated, and interactive visualizations.
- Advantages: Highly customizable, and supports a wide range of plot types.
- Applications: Basic plots (line, bar, scatter), complex multi-plot layouts.
- Seaborn: Built on top of Matplotlib, provides a high-level interface for drawing attractive statistical graphics.
- Advantages: Simplifies the creation of complex plots, and includes built-in themes and color palettes.
- Applications: Statistical plots (box plots, violin plots, pair plots).
Tableau
- Description: A powerful business intelligence tool for creating interactive and shareable dashboards.
- Features: Drag-and-drop interface, supports numerous data sources, real-time analytics.
- Applications: Business reporting, data analysis, interactive dashboard creation.
Power BI
- Description: Microsoft’s interactive data visualization and business intelligence tool.
- Features: Integration with other Microsoft products, real-time data access, and robust sharing capabilities.
- Applications: Business analytics, report generation, KPI tracking.
Effective Communication
Storytelling with Data
- Description: The practice of crafting a narrative around data to make insights clear and engaging.
- Techniques: Using clear visuals, providing context, and emphasizing key points.
- Benefits: Enhances understanding, aids decision-making, and captures audience interest.
Report Writing
- Tools: Jupyter Notebooks, R Markdown.
- Content: Clear explanation of analysis, visualizations, results, and conclusions.
- Advantages: Facilitates reproducibility, combines code and narrative, and supports various output formats (HTML, PDF).
Presentations
- Tools: PowerPoint, Google Slides, Prezi.
- Techniques: Use of visuals, concise text, and storytelling.
- Benefits: Effectively communicate findings to non-technical stakeholders, and support decision-making processes.
Interactive Visualizations
Plotly and Bokeh
- Plotly: An open-source graphing library for interactive, web-based data visualizations.
- Features: Supports a wide range of chart types, and integrates with web frameworks.
- Applications: Interactive dashboards, web applications.
- Bokeh: A Python library for creating interactive visualizations for modern web browsers.
- Features: High-performance interactivity, and real-time streaming.
- Applications: Data applications, interactive plots.
Dash
- Description: A framework for building web applications with Python, utilizing Plotly for interactive graphs.
- Features: Integrates with Flask, and supports complex user interfaces.
- Applications: Data-driven web applications, interactive dashboards.
Big Data Technologies: Hadoop, Spark, and NoSQL Databases
Hadoop
HDFS (Hadoop Distributed File System)
- Description: A distributed file system designed to run on commodity hardware, providing high throughput access to data.
- Features: Fault tolerance, scalability, handles large datasets.
- Applications: Large-scale data storage, data-intensive computations.
MapReduce
- Description: A programming model for processing large datasets in parallel across a distributed cluster.
- Components: Map function processes input data, reduce function aggregates results.
- Applications: Data processing tasks like sorting, filtering, and summarization.
YARN (Yet Another Resource Negotiator)
- Description: A resource management layer for Hadoop, allowing multiple data processing engines to handle data stored in a single platform.
- Features: Resource allocation, job scheduling.
- Applications: Manages resources for different Hadoop applications, and improves cluster utilization.
Spark
In-Memory Computing
- Description: Processes data in memory to enhance the speed of data processing tasks compared to disk-based systems.
- Advantages: Significant performance improvements for iterative algorithms and interactive data analysis.
Components
- Spark SQL: A module for structured data processing using SQL queries.
- Spark Streaming: Enables scalable, high-throughput, fault-tolerant stream processing.
- MLlib: A machine learning library providing various algorithms and utilities.
- GraphX: A library for graph computation and analysis.
Resilient Distributed Datasets (RDDs)
- Description: Immutable, distributed collections of objects that can be processed in parallel.
- Features: Fault-tolerant, supports in-memory computing.
- Applications: Foundation for data-parallel operations in Spark.
NoSQL Databases
MongoDB
- Description: A document-oriented NoSQL database, storing data in flexible, JSON-like documents.
- Features: Schema flexibility, horizontal scalability, high availability.
- Applications: Content management, real-time analytics, IoT applications.
Cassandra
- Description: A wide-column store NoSQL database designed for handling large amounts of data across many servers.
- Features: Decentralized, fault-tolerant, linear scalability.
- Applications: Log management, recommendation systems, real-time analytics.
HBase
- Description: A distributed, scalable big data store modeled after Google’s Big Table, written in Java.
- Features: Strong consistency, support for large tables, scalable.
- Applications: Real-time data processing, analytics on large datasets, time-series data.
By mastering these advanced skills in machine learning, artificial intelligence, data visualization, and big data technologies, professionals can effectively analyze complex datasets, build predictive models, and communicate insights, driving data-driven decision-making across various industries.
Educational Resources and Training
University Courses and Specialized Programs
- Traditional Degree Programs:
- Bachelor’s Degree: Provides foundational knowledge and skills in a chosen field. Typically takes 3-4 years to complete. Courses include lectures, lab work, and projects.
- Master’s Degree: Offers advanced education and specialization in a specific area. Usually 1-2 years of study involving coursework, research, and a thesis or capstone project.
- Doctoral Degree (Ph.D.): Focuses on original research, advancing knowledge in a particular field. It typically spans 3-7 years and culminates in a dissertation.
- Specialized Programs:
- Professional Certificates: Short-term programs that provide specialized training and skills in specific areas such as data science, cybersecurity, or project management.
- Executive Education: Tailored for professionals looking to enhance their skills in leadership, management, and industry-specific expertise. Often provided by business schools.
Online Learning Platforms and MOOCs
- Massive Open Online Courses (MOOCs):
- Coursera: Offers courses from universities like Stanford, Yale, and others. Covers a wide range of subjects including computer science, business, and humanities.
- edX: Provides access to courses from institutions such as MIT, Harvard, and Berkeley. Includes free courses with options to pay for certificates.
- Udacity: Focuses on technology and career-focused courses like AI, programming, and data analysis.
- Subscription-Based Platforms:
- LinkedIn Learning: Provides a vast library of video tutorials on topics like business, technology, and creative skills. Available via subscription.
- Pluralsight: Offers tech-focused courses, particularly in software development, IT operations, and cybersecurity.
- Specialized Online Programs:
- Skillshare: Emphasizes creative skills and entrepreneurship. Offers classes in design, writing, photography, and more.
- Khan Academy: Provides free educational resources primarily for K-12 education but also covers some higher education subjects.
Workshops, Bootcamps, and Certification Programs
- Intensive Bootcamps:
- Coding Bootcamps: Programs like General Assembly, Flatiron School, and Le Wagon offer intensive training in software development, web development, and data science. Typically last 12-24 weeks.
- Data Science Bootcamps: Institutions like DataCamp and Springboard provide focused training on data analysis, machine learning, and data visualization.
- Workshops:
- Short-Term Workshops: Conducted by industry experts, these workshops focus on specific skills or topics such as digital marketing, UI/UX design, or advanced Excel techniques.
- Corporate Training Workshops: Tailored for businesses, these workshops help employees upskill in areas critical to their roles.
- Certification Programs:
- Professional Certifications: Certifications like PMP (Project Management Professional), CISSP (Certified Information Systems Security Professional), and CPA (Certified Public Accountant) validate expertise and are often required for advanced positions.
- Vendor-Specific Certifications: Offered by companies like Microsoft, Cisco, and AWS, these certifications validate skills in using specific technologies and platforms.
Practical Experience and Projects
Internships and Industry Collaborations
- Internships:
- Summer Internships: Typically lasting 8-12 weeks, these provide hands-on experience in a professional setting, often leading to job offers.
- Co-Op Programs: Alternating periods of academic study and work experience, providing in-depth exposure to industry practices and potential job placements.
- Industry Collaborations:
- University-Industry Partnerships: Collaborative projects between academic institutions and businesses, offering students real-world problems to solve.
- Corporate Sponsorships: Companies fund academic research or projects, providing resources and industry insights.
Academic Research and Publications
- Research Projects:
- Undergraduate Research: Opportunities for students to engage in research early in their academic careers, often through research assistant positions or special programs.
- Graduate Research: Integral to Master’s and Ph.D. programs, involving in-depth study and original contributions to the field.
- Publications:
- Journals and Conferences: Publishing research findings in peer-reviewed journals and presenting at conferences to disseminate knowledge and gain professional recognition.
- Collaborative Publications: Working with faculty or industry professionals on joint research papers enhances learning and professional networks.
Hackathons, Competitions, and Open Source Contributions
- Hackathons:
- Student Hackathons: Events where students collaboratively build projects over short periods, fostering innovation and practical problem-solving skills.
- Corporate-Sponsored Hackathons: Companies host hackathons to identify talent and innovative solutions, offering prizes and potential job opportunities.
- Competitions:
- Academic Competitions: Challenges like math Olympiads, engineering contests, and case study competitions help develop analytical and problem-solving skills.
- Professional Competitions: Events like coding challenges (e.g., Google Code Jam), cybersecurity competitions (e.g., Capture the Flag), and design contests.
- Open Source Contributions:
- Open Source Projects: Contributing to projects on platforms like GitHub allows individuals to collaborate globally, improve coding skills, and build a portfolio.
- Community Involvement: Participating in open-source communities provides networking opportunities, mentorship, and a chance to work on impactful projects.
By leveraging these educational resources and engaging in practical experiences, individuals can enhance their knowledge, skills, and professional development, positioning themselves for successful careers in their chosen fields.
Role of Industry and Government Initiatives
Industry-Academia Partnerships
- Industry-academia partnerships play a crucial role in bridging the gap between academic knowledge and practical application in the field of data science.
- These partnerships often involve collaboration between universities or research institutions and corporations or businesses operating in various sectors.
- Through these partnerships, academia gains access to real-world data sets, industry expertise, and cutting-edge technologies, while industry partners benefit from the latest research findings, fresh perspectives, and access to a pool of talented graduates.
- Collaborative projects, joint research initiatives, and sponsored programs are common forms of industry-academia partnerships in data science.
- These partnerships not only facilitate knowledge transfer but also contribute to the development of innovative solutions to real-world problems.
Government Programs and Policies Supporting Data Science Education
- Governments around the world recognize the growing importance of data science in driving economic growth, innovation, and societal progress. As a result, many governments have implemented programs and policies to support data science education at various levels.
- These programs may include funding for research projects, grants for educational institutions, scholarships for students pursuing degrees in data science-related fields, and initiatives to develop standardized curricula.
- Government agencies also collaborate with industry partners and academic institutions to design and implement initiatives aimed at enhancing data science education and training.
- Additionally, governments may invest in infrastructure and resources to facilitate the teaching and learning of data science, such as establishing data science labs, providing access to computational resources, and organizing workshops and seminars.
Corporate Training and Development Programs
- In response to the growing demand for data science skills in the workforce, many corporations have developed training and development programs to upskill their employees and prepare them for the challenges of the digital age.
- These programs may include both formal training courses and informal learning opportunities, such as workshops, webinars, online tutorials, and hands-on projects.
- Corporate training programs often cover a wide range of topics in data science, including statistical analysis, machine learning, data visualization, and big data technologies.
- Employers may also offer certification programs or reimbursement for employees seeking to obtain professional certifications in data science.
- By investing in the continuous learning and development of their workforce, companies can ensure that they remain competitive in the rapidly evolving field of data science and analytics.
Challenges and Solutions in Mastering Data Science
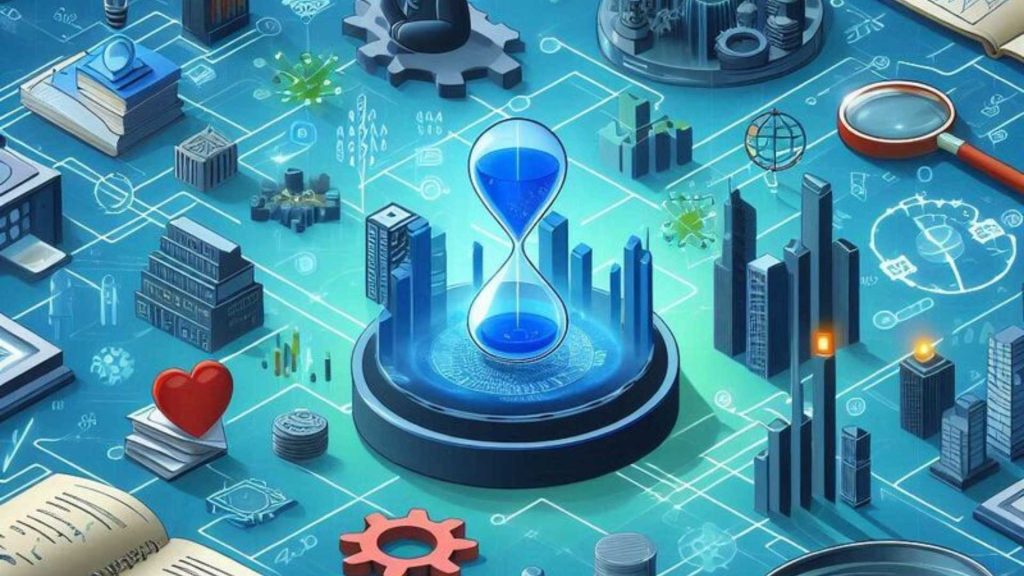
Balancing Theory and Practical Application
- One of the key challenges in mastering data science is striking the right balance between theoretical knowledge and practical skills.
- While a solid understanding of underlying concepts and algorithms is essential, it is equally important for data scientists to have hands-on experience working with real-world data sets and solving practical problems.
- To address this challenge, educational institutions and training providers can incorporate project-based learning, case studies, and internships into their curriculum, allowing students to apply theoretical concepts to real-world scenarios.
- Employers can also provide opportunities for employees to work on challenging projects and collaborate with cross-functional teams to gain practical experience and enhance their problem-solving skills.
Keeping Up with Rapid Technological Changes
- Another major challenge in mastering data science is keeping pace with the rapid technological advancements in the field.
- New tools, techniques, and algorithms are constantly being developed, making it difficult for data scientists to stay updated with the latest trends and best practices.
- To overcome this challenge, professionals in the field must adopt a lifelong learning mindset and actively seek out opportunities to expand their knowledge and skills.
- Continuous professional development through online courses, workshops, conferences, and networking events can help data scientists stay abreast of emerging technologies and trends.
- Employers can also support their employees’ professional development by providing access to training resources, encouraging participation in industry events, and fostering a culture of innovation and experimentation within the organization.
Addressing Skill Gaps and Continuous Learning
- Skill gaps are another challenge that data scientists often face, especially as the field continues to evolve and diversify.
- These skill gaps may arise from changes in technology, shifts in industry demands, or gaps in traditional education and training programs.
- To address skill gaps, individuals can take proactive steps to identify areas for improvement and seek out targeted learning opportunities to fill those gaps.
- Employers can also play a role in addressing skill gaps by providing tailored training and development programs, offering mentorship and coaching, and creating opportunities for employees to gain exposure to new technologies and methodologies.
- Additionally, collaboration between industry, academia, and government can help ensure that educational programs and training initiatives are aligned with the evolving needs of the data science workforce, enabling professionals to acquire the skills they need to succeed in their careers.
Case Studies and Success Stories
- Profile of Successful Data Scientists from Indian Engineering Backgrounds
- Introduction: Highlight the prevalence of Indian engineers in the field of data science.
- Case Studies:
- Case 1: A graduate from an Indian engineering college who transitioned into data science and now works at a leading tech firm.
- Case 2: A story of a data scientist who started their career in India and went on to make significant contributions in the field globally.
- Success Factors:
- Educational Background: Discuss how engineering education provides a strong foundation for data science.
- Skill Development: Highlight the importance of continuous learning and upskilling in this dynamic field.
- Networking and Opportunities: Emphasize the role of networking and seizing opportunities for career growth.
- Lessons Learned: Summarize key takeaways from the success stories and discuss how aspiring data scientists can emulate their paths.
Impact of Data Science Projects on Industry and Society
- Introduction: Discuss the growing influence of data science projects on various sectors.
- Industry Impact:
- Improved Efficiency: Explore how data science optimizes processes and decision-making in industries like finance, healthcare, and retail.
- Enhanced Customer Experience: Highlight examples of how data-driven insights lead to better products and services.
- Cost Reduction: Discuss how predictive analytics and machine learning algorithms help in cost-saving measures.
- Societal Impact:
- Healthcare: Discuss the role of data science in disease prediction, personalized medicine, and public health initiatives.
- Environment: Explore how data science contributes to environmental monitoring, conservation efforts, and climate change research.
- Education: Highlight the use of data analytics in personalized learning, adaptive assessments, and educational research.
- Ethical Considerations: Address the ethical implications of data science projects, such as privacy concerns, bias in algorithms, and data security.
Lessons Learned and Best Practices
- Continuous Learning: Emphasize the importance of staying updated with the latest technologies and methodologies in data science.
- Problem-Solving Skills: Discuss how developing strong problem-solving abilities can enhance a data scientist’s effectiveness.
- Collaboration: Highlight the value of interdisciplinary collaboration and teamwork in tackling complex data science projects.
- Communication Skills: Stress the significance of effectively communicating insights and findings to stakeholders with varying levels of technical expertise.
- Quality Assurance: Discuss the importance of rigorous testing and validation to ensure the accuracy and reliability of data science models.
Future Trends and Emerging Technologies
- Advancements in Machine Learning and AI
- Introduction to the rapid evolution of machine learning and AI technologies.
- Deep Learning: Discuss advancements in neural networks, such as transformer models and GANs.
- Reinforcement Learning: Explore applications of reinforcement learning in areas like robotics and game theory.
- AI Ethics: Address the growing focus on ethical AI development and the integration of fairness, transparency, and accountability into AI systems.
- Role of Data Science in Industry 4.0
- Overview of Industry 4.0 and its emphasis on automation, IoT, and data-driven decision-making.
- Smart Manufacturing: Discuss how data science enables predictive maintenance, supply chain optimization, and real-time monitoring in manufacturing settings.
- Digital Twins: Explore the concept of digital twins and their role in simulating and optimizing processes in various industries.
- Cyber-Physical Systems: Highlight the integration of data science with physical systems to enhance efficiency and productivity.
- Ethical Considerations and Responsible AI
- Introduction to the ethical challenges posed by AI and data science.
- Bias and Fairness: Discuss strategies for mitigating bias in algorithms and ensuring fairness in decision-making processes.
- Privacy and Security: Address concerns related to data privacy, cybersecurity, and the responsible use of personal data.
- Transparency and Accountability: Highlight the importance of transparent AI systems and mechanisms for holding AI developers accountable for their creations.
Conclusion
In summarizing our exploration of the intersection between engineering education and the burgeoning field of data science, it becomes evident that Indian engineering students stand at a critical juncture with immense potential for growth and opportunity. By equipping themselves with data science skills, they can unlock a myriad of possibilities in various industries and contribute significantly to India’s technological advancement.
Summary of Key Points
- Importance of Data Science Skills: Engineering students must recognize the significance of data science proficiency in today’s digital age, where data-driven decision-making is paramount.
- Current Landscape in India: The data science landscape in India is burgeoning, with a growing demand for skilled professionals across sectors such as IT, finance, healthcare, and e-commerce.
- Challenges Faced: Despite the vast opportunities, engineering students encounter challenges such as lack of exposure, limited resources, and competition from non-engineering backgrounds.
- Opportunities Available: However, numerous opportunities exist for Indian engineers in data science roles, ranging from data analysts to machine learning engineers, presenting avenues for career advancement and innovation.
The Road Ahead for Indian Engineering Students in Data Science
- Strategies for Skill Development: Engineering students should focus on acquiring a strong foundation in mathematics, statistics, and programming languages like Python and R, supplemented by hands-on experience with data analysis tools and techniques.
- Networking and Internship Opportunities: Actively participating in industry events, and workshops, and securing internships can provide invaluable exposure and networking opportunities.
- Academic and Industry Collaboration: Universities and industry stakeholders should collaborate to design a curriculum that aligns with industry needs, offering practical training and internships to bridge the gap between academia and industry.
- Continuous Learning and Upskilling: Lifelong learning is crucial in the rapidly evolving field of data science, necessitating a commitment to continuous upskilling through online courses, certifications, and participation in community-driven projects.
Final Thoughts and Recommendations
- Encouragement for Perseverance: Engineering students embarking on a data science journey should remain resilient in the face of challenges, leveraging setbacks as learning opportunities to propel their growth.
- Importance of Adaptability: In a dynamic field like data science, adaptability is key. Engineers should embrace change, stay abreast of emerging technologies, and be willing to pivot their skill sets accordingly.
- Seeking Mentorship and Guidance: Mentorship from seasoned professionals can provide invaluable insights, guidance, and support in navigating the complexities of a data science career.
- Call to Action for Proactive Career Planning: Indian engineering students are urged to take proactive steps in charting their career path, setting clear goals, and leveraging available resources to realize their aspirations in the data science domain.
Trizula Digital Solutions presents an exclusive opportunity for IT students to delve into the realm of data science through our comprehensive data science full course. Tailored to match academic pursuits, our self-paced curriculum ensures industry readiness upon graduation, covering vital aspects like Python, AI, ML, and NLP. Elevate your skills and secure your professional future today!
Embark on a transformative journey with Trizula Mastery in Data Science, designed to empower engineering students with cutting-edge competencies vital for today’s job market. Our flexible approach ensures seamless integration with academic commitments while fostering proficiency in data science essentials. Don’t miss out on this chance to shape your career trajectory – Click Here Now and kickstart your journey toward success!
Data Science Skills FAQs: Common Queries Answered:
1. Which skill is required for data science?
Data science requires a combination of skills including programming, statistics, machine learning, data visualization, and domain knowledge.
2. Does data science require coding skills?
Yes, coding skills are essential for data science, particularly proficiency in programming languages such as Python, R, and SQL.
3. Where can I practice data science skills?
You can practice data science skills through online platforms like Kaggle, DataCamp, and Coursera, and by working on real-world projects, participating in hackathons, or contributing to open-source projects.
4. How can I improve my data science skills?
You can improve your data science skills by consistently practicing coding, learning new algorithms and techniques, working on diverse datasets, seeking mentorship, and staying updated with industry trends.
5. Is data science a valuable skill?
Yes, data science is a highly valuable skill in today’s data-driven world, with increasing demand across various industries for professionals who can derive actionable insights from data to drive decision-making and innovation.