Target Audience: Students pursuing degrees in statistics, data science, or related fields like Statistical Tools. Professionals working in industries that rely on data analysis and decision-making. Anyone interested in learning about statistical tools and techniques for personal or professional development
Value Proposition:
- Gain a comprehensive understanding of the fundamental statistical tools and techniques used in data analysis
- Learn how to apply these methods to real-world problems and make informed decisions based on data
- Develop skills that are highly valued in the job market and can lead to career advancement opportunities
Key Takeaways:
- Understand the importance of statistical tools and techniques in data analysis and decision-making processes
- Become familiar with the various types of statistical tests and techniques, including descriptive statistics, inferential statistics, and multivariate analysis
- Learn how to design experiments, analyze time series data, and use statistical software and tools effectively
- Explore the applications of statistical methods across different industries and the ethical considerations involved in statistical analysis
- Gain the knowledge and skills necessary to pursue further studies or excel in careers related to data analysis and statistics
Statistical Tools and Techniques: An Introductory Overview
Statistical tools are mathematical instruments used to collect, analyze, interpret, and present data. They are fundamental in transforming raw data into meaningful insights, enabling researchers and analysts to make informed decisions. These tools encompass a wide range of methods and software that streamline data processing and enhance accuracy.
- Definition: Statistical tools refer to the techniques and software used for data collection, analysis, interpretation, and presentation.
- Importance: They help in understanding data patterns, testing hypotheses, and making predictions.
- Applications: Used across various fields like business, healthcare, social sciences, and more to derive actionable insights.
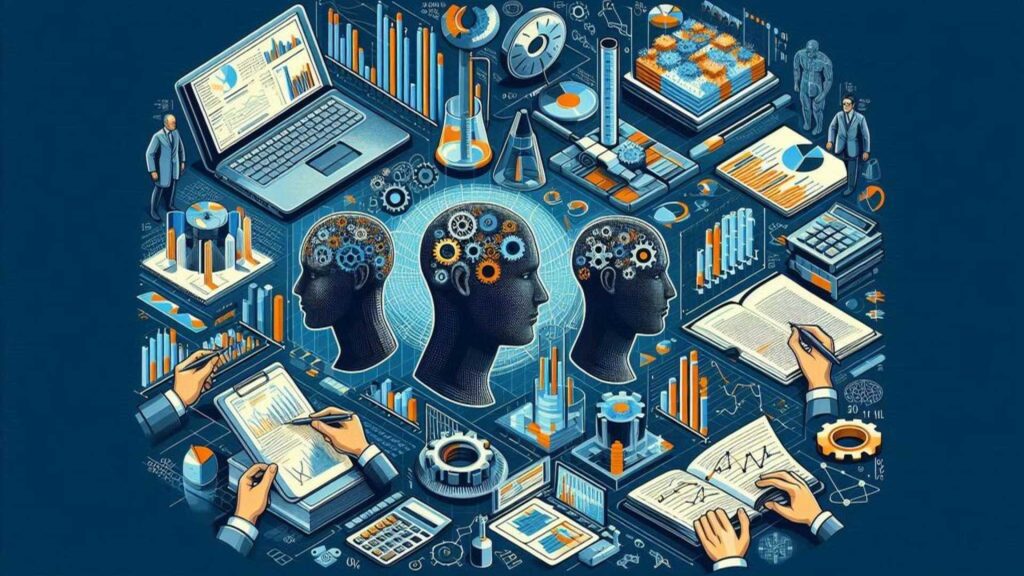
Statistical tools are essential for students across various fields, as they provide the necessary skills to collect, analyze, interpret, and present data effectively. These tools enable students to transform raw data into meaningful insights, make informed decisions, understand data patterns, test hypotheses, and make predictions. By mastering statistical tools, students can enhance their analytical abilities and gain a competitive edge in their academic and professional pursuits.
Role of Statistical Techniques in Decision-Making Processes
Statistical techniques play a crucial role in decision-making by providing a scientific basis for analyzing data and making predictions. They help organizations to identify trends, test hypotheses, and measure uncertainty, leading to more informed and effective decisions.
- Trend Analysis: Identifying patterns and trends over time to guide strategic planning.
- Hypothesis Testing: Validating assumptions and theories with statistical evidence.
- Uncertainty Measurement: Assessing risks and probabilities to make more robust decisions.
Statistical techniques are invaluable tools for students in their academic and professional pursuits. By understanding and applying these techniques, students can gain a scientific basis for analyzing data, identifying trends, testing hypotheses, and measuring uncertainty, ultimately leading to more informed and effective decision-making. These skills are crucial for students across various disciplines, from business and economics to the sciences and social sciences, as they navigate their studies, research projects, and future career paths.
Descriptive Statistics
Descriptive statistics involve summarizing and describing the main features of a dataset. They provide a concise overview of the data, including measures of central tendency (mean, median, mode), measures of variability (range, variance, standard deviation), and visual representations (graphs, charts).
Measures of Central Tendency (Mean, Median, Mode)
Measures of central tendency are used to describe the center of a data set. They provide a single value that represents the entire distribution, helping to understand the typical value around which the data clusters.
- Mean: The arithmetic average of a data set.
- Calculation: Sum of all values divided by the number of values.
- Use Case: Ideal for normally distributed data without outliers.
- Median: The middle value when data is ordered.
- Calculation: The central number in an ordered data set, or the average of the two central numbers if the data set has an even number of values.
- Use Case: Effective for skewed distributions or data with outliers.
- Mode: The most frequently occurring value in a data set.
- Calculation: The value that appears most often.
- Use Case: Useful for categorical data or data with repeated values.
Measures of Dispersion (Range, Variance, Standard Deviation)
Measures of dispersion provide insights into the variability or spread of data points in a dataset. They help to understand how much the data deviates from the central tendency.
- Range: The difference between the highest and lowest values.
- Calculation: Maximum value minus minimum value.
- Use Case: Simple measure of variability but sensitive to outliers.
- Variance: The average of the squared differences from the mean.
- Calculation: Sum of squared deviations from the mean divided by the number of observations.
- Use Case: Indicates how spread out the data points are.
- Standard Deviation: The square root of the variance.
- Calculation: The square root of the average squared differences from the mean.
- Use Case: Provides a measure of spread in the same units as the data.
Frequency Distributions and Graphical Representations
Frequency distributions show how often each value in a data set occurs, and graphical representations visually summarize data for easier interpretation.
- Frequency Distribution: A table that displays the frequency of various outcomes in a sample.
- Construction: List data values and their corresponding frequencies.
- Use Case: Helps in understanding the distribution of data.
- Histograms: Bar graphs representing the frequency distribution of a continuous data set.
- Features: Bars show the frequency of intervals.
- Use Case: Ideal for visualizing the shape of data distribution.
- Box Plots: Graphical depiction of data through their quartiles.
- Features: Shows minimum, first quartile, median, third quartile, and maximum.
- Use Case: Useful for identifying outliers and understanding data spread.
Measures of central tendency, such as mean, median, and mode, provide students with a concise summary of the typical values in a dataset, while measures of dispersion, including range, variance, and standard deviation, help students understand the variability and spread of the data. Frequency distributions and graphical representations, such as histograms and box plots, enable students to visually interpret and analyze the distribution of data, equipping them with essential skills for data-driven decision-making and problem-solving.
Inferential Statistics
Inferential statistics involve drawing conclusions and making inferences about a population based on a sample. These techniques allow students to test hypotheses, estimate parameters, and assess the significance of relationships, enabling them to make informed decisions and predictions.
Probability Concepts (Random Variables, Probability Distributions)
Probability concepts form the foundation of inferential statistics, allowing analysts to make predictions and inferences about a population based on sample data.
- Random Variables: Variables that can take on different values due to chance.
- Types: Discrete (specific values) and continuous (any value within a range).
- Use Case: Modeling real-world uncertainties.
- Probability Distributions: Functions that describe the likelihood of different outcomes.
- Types: Normal, binomial, Poisson, etc.
- Use Case: Determining probabilities of various outcomes.
Sampling Methods and Techniques (Simple Random Sampling, Stratified Sampling, etc.)
Sampling methods are techniques used to select a subset of data from a larger population, allowing for analysis and inference about the population.
- Simple Random Sampling: Every member of the population has an equal chance of being selected.
- Process: Randomly selecting individuals from the population.
- Use Case: Ensures unbiased representation.
- Stratified Sampling: The population is divided into strata, and random samples are taken from each stratum.
- Process: Identifying strata and then randomly sampling within each.
- Use Case: Ensures representation of all subgroups.
- Cluster Sampling: Dividing the population into clusters and randomly selecting entire clusters.
- Process: Grouping the population into clusters and sampling some clusters.
- Use Case: Cost-effective and practical for large populations.
Estimation (Confidence Intervals) and Hypothesis Testing
Estimation involves using sample data to estimate population parameters, while hypothesis testing assesses the validity of assumptions or claims about a population.
- Confidence Intervals: Range within which a population parameter is expected to lie with a certain level of confidence.
- Calculation: Sample mean ± margin of error.
- Use Case: Provides an estimate of the population parameter with an associated confidence level.
- Hypothesis Testing: Process of testing assumptions about a population parameter.
- Steps: Formulate null and alternative hypotheses, select the significance level, calculate the test statistic, and make a decision based on the p-value.
- Use Case: Validates or refutes assumptions using sample data.
Probability concepts, such as random variables and probability distributions, provide a foundation for inferential statistics, allowing students to model uncertainties and determine probabilities of various outcomes. Sampling methods, including simple random sampling, stratified sampling, and cluster sampling, enable students to select representative subsets of data for analysis, while estimation techniques, such as confidence intervals, and hypothesis testing help students make inferences about population parameters and validate assumptions using sample data.
Statistical Tests and Techniques
Statistical tests and techniques, such as t-tests, ANOVA, regression analysis, and correlation analysis, allow students to identify relationships, compare groups, and make predictions based on data. These methods equip students with the tools to draw meaningful insights and make informed decisions.
Parametric vs. Non-Parametric Tests
Statistical tests can be broadly categorized into parametric and non-parametric tests, depending on the assumptions they make about the data distribution. Parametric tests assume underlying statistical distributions, while non-parametric tests do not, making them suitable for data that do not meet parametric assumptions.
- Parametric Tests:
- Assume specific distribution, typically normal.
- Require knowledge of population parameters (mean, standard deviation).
- Examples: t-tests, ANOVA, regression analysis.
- Non-Parametric Tests:
- Do not assume specific data distribution.
- Suitable for ordinal data or non-normally distributed interval/ratio data.
- Examples: Mann-Whitney U test, Kruskal-Wallis test, Chi-square test.
Commonly Used Statistical Tests
t-tests
t-tests are used to compare the means of two groups to determine if they are significantly different from each other. This test is applicable when the data follows a normal distribution and the samples are independent.
- Types of t-tests:
- One-sample t-test: Compares the sample mean to a known value or population mean.
- Independent two-sample t-test: Compares the means of two independent groups.
- Paired sample t-test: Compares means from the same group at different times.
- Applications:
- Comparing average test scores between two different classes.
- Evaluating the effect of a treatment by comparing pre-treatment and post-treatment measurements.
ANOVA (Analysis of Variance)
ANOVA is a statistical method used to compare the means of three or more groups to see if at least one of them is significantly different. It extends the t-test to multiple groups.
- Types of ANOVA:
- One-way ANOVA: Compares means across one independent variable.
- Two-way ANOVA: Compares means with two independent variables, allowing interaction effects.
- Repeated measures ANOVA: Used when the same subjects are used for each treatment.
- Applications:
- Comparing sales performance across multiple regions.
- Testing the effectiveness of different marketing strategies on sales.
Chi-square Test
The Chi-square test is used to determine if there is a significant association between two categorical variables. It compares the observed frequencies in each category to the expected frequencies if there are no associations.
- Types of Chi-square tests:
- Chi-square goodness-of-fit test: Determines if a sample matches a population.
- Chi-square test of independence: Assesses if two categorical variables are independent.
- Applications:
- Testing if gender is independent of voting preference.
- Evaluating if there is a preference for different brands of a product.
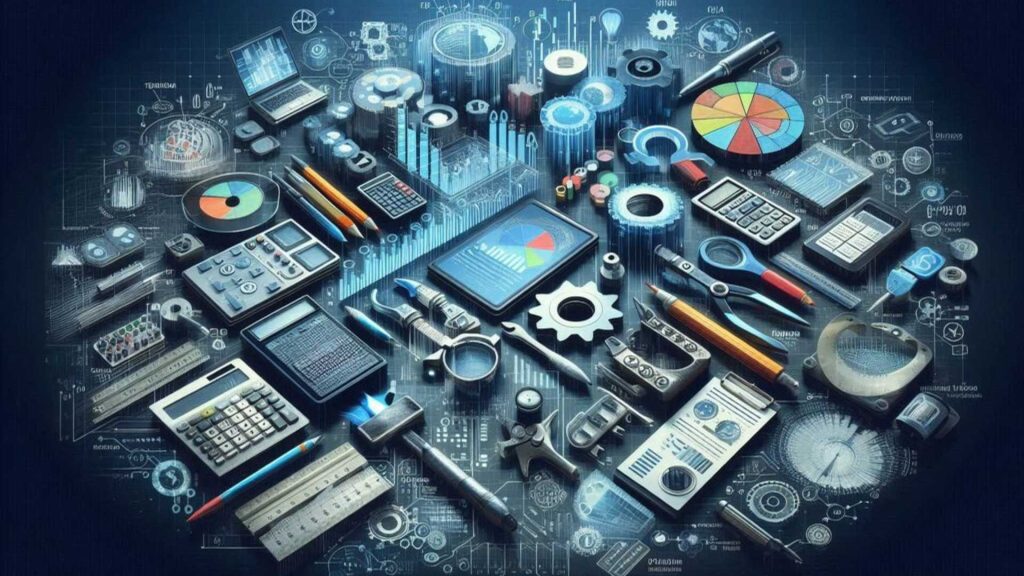
Correlation Analysis
Correlation analysis measures the strength and direction of the relationship between two continuous variables. It quantifies how changes in one variable are associated with changes in another.
- Types of correlation:
- Pearson correlation: Measures linear relationship for normally distributed data.
- Spearman rank correlation: Measures monotonic relationship for non-normally distributed data.
- Applications:
- Examining the relationship between hours studied and exam scores.
- Analyzing the correlation between advertising spend and sales revenue.
Regression Analysis
Regression analysis is used to model the relationship between a dependent variable and one or more independent variables. It helps in predicting the value of the dependent variable based on the values of the independent variables.
- Types of regression:
- Linear regression: Models linear relationships between variables.
- Logistic regression: Models binary outcomes.
- Multiple regression: Models relationships with multiple independent variables.
- Applications:
- Predicting house prices based on features like size, location, and age.
- Modeling the probability of customer churn based on usage patterns.
Understanding the differences between parametric and non-parametric tests is crucial for students to select appropriate statistical methods for their research, enabling them to draw valid conclusions from their data. Parametric tests, such as t-tests, ANOVA, and regression analysis, assume specific data distributions and require knowledge of population parameters, while non-parametric tests, including the Mann-Whitney U test, Kruskal-Wallis test, and Chi-square test, do not make assumptions about the underlying distribution and are suitable for ordinal data or non-normally distributed interval/ratio data.
Experimental Design
Experimental design involves planning and structuring experiments to ensure the validity and reliability of results. It helps students minimize bias, control for confounding variables, and maximize the statistical power of their studies, leading to more robust and meaningful conclusions.
Principles of Experimental Design
Experimental design involves planning experiments to ensure that the data obtained can be analyzed to yield valid and objective conclusions. Key principles include randomization, replication, and blocking.
- Randomization: Randomly assigning subjects to different groups to eliminate bias.
- Replication: Repeating the experiment to ensure reliability and generalizability of results.
- Blocking: Grouping similar experimental units to reduce variability.
Control Groups and Experimental Variables
Control groups are essential in experiments to establish a baseline for comparison. Experimental variables are manipulated to observe their effects on the outcome variable.
- Control Group: A group that does not receive the treatment, used as a benchmark.
- Experimental Variables: Independent variables manipulated in the experiment.
- Dependent Variables: The outcome variables measured in the experiment.
Factorial Designs and Randomized Controlled Trials (RCTs)
Factorial designs and RCTs are advanced experimental designs that allow researchers to investigate multiple factors simultaneously and establish cause-and-effect relationships.
- Factorial Designs:
- Definition: Experimental setups where all possible combinations of factors and levels are investigated.
- Advantages: Efficiently evaluates the effect of multiple factors and their interactions.
- Applications: Agricultural experiments, marketing strategy evaluations.
- Randomized Controlled Trials (RCTs):
- Definition: Participants are randomly assigned to treatment or control groups to test the efficacy of interventions.
- Advantages: Gold standard for establishing causality, minimizes biases.
- Applications: Clinical trials, policy impact assessments.
Experimental design principles, such as randomization, replication, and blocking, help students plan and structure experiments to ensure the validity and reliability of results, while control groups and experimental variables allow students to establish baselines, manipulate factors, and measure outcomes. Factorial designs and randomized controlled trials (RCTs) enable students to investigate multiple factors simultaneously and establish cause-and-effect relationships, providing a robust framework for conducting rigorous and impactful research.
Multivariate Analysis
Multivariate analysis involves the simultaneous analysis of multiple variables to understand complex relationships and patterns in data. It includes techniques like multiple regression, factor analysis, and cluster analysis, allowing students to gain deeper insights and make more informed decisions.
Principal Component Analysis (PCA)
PCA is a dimensionality reduction technique used to transform high-dimensional data into a lower-dimensional form, preserving as much variability as possible.
- Key Concepts:
- Eigenvalues and Eigenvectors: PCA uses these to identify the principal components.
- Variance Explained: Each principal component accounts for a portion of the total variance.
- Applications: Reducing the dimensionality of large datasets, visualizing data in 2D/3D.
- Steps in PCA:
- Standardize the data.
- Compute the covariance matrix.
- Calculate eigenvalues and eigenvectors.
- Select principal components and transform the data.
Factor Analysis
Factor analysis is a technique used to identify underlying factors or constructs that explain the patterns of correlations among observed variables.
- Types of Factor Analysis:
- Exploratory Factor Analysis (EFA): Identifies the underlying factor structure without preconceived notions.
- Confirmatory Factor Analysis (CFA): Tests hypotheses about the factor structure based on theoretical expectations.
- Applications:
- Psychometrics: Identifying latent constructs like intelligence, and anxiety.
- Market research: Understanding consumer preferences and behaviors.
Cluster Analysis
Cluster analysis is a technique used to group similar objects into clusters based on their characteristics. It helps in identifying natural groupings in the data.
- Types of Clustering:
- Hierarchical Clustering: Builds a tree-like structure of nested clusters.
- K-means Clustering: Partitions data into K clusters based on the nearest mean.
- DBSCAN: Density-based clustering that identifies clusters of varying shapes and sizes.
- Applications:
- Customer segmentation in marketing.
- Image recognition and classification.
Discriminant Analysis
Discriminant analysis is used to classify observations into predefined groups based on predictor variables. It helps in understanding the differences between groups and predicting group membership.
- Types of Discriminant Analysis:
- Linear Discriminant Analysis (LDA): Assumes linear relationships and equal covariance matrices across groups.
- Quadratic Discriminant Analysis (QDA): Allows for different covariance matrices, suitable for non-linear boundaries.
- Applications:
- Credit scoring: Classifying loan applicants as high or low risk.
- Medical diagnosis: Predicting disease presence based on clinical measurements.
Principal component analysis (PCA) helps students reduce the dimensionality of large datasets while preserving variability, enabling better visualization and understanding of complex data structures. Factor analysis identifies underlying constructs that explain patterns in observed variables, cluster analysis groups similar objects based on their characteristics, and discriminant analysis classifies observations into predefined groups based on predictor variables, providing students with powerful tools for exploring and understanding multivariate relationships in their research.
Time Series Analysis
Time series analysis involves the study of data collected over time to identify patterns, trends, and relationships. It includes techniques like moving averages, exponential smoothing, and ARIMA models, allowing students to make forecasts, detect anomalies, and gain insights into temporal dynamics.
Components of Time Series Data
Time series data consists of observations recorded sequentially over time. Key components of time series data include trend, seasonality, cycles, and irregularities. Understanding these components is essential for effective analysis and forecasting.
- Trend: The long-term progression of the series, indicates a general direction in the data over time.
- Seasonality: Regular, repeating patterns or fluctuations observed within a fixed period, such as daily, monthly, or yearly.
- Cycles: Longer-term oscillations that may not have a fixed period, often related to economic or business cycles.
- Irregularities: Random, unpredictable variations or noise that do not fit into the trend, seasonal, or cyclical components.
Forecasting Techniques
Forecasting time series data involves using historical data to predict future values. Several techniques can be employed, ranging from simple methods to complex models.
- Moving Averages: Smooth out short-term fluctuations to highlight longer-term trends. Suitable for data with no strong seasonality or trend.
- Simple Moving Average: Average of the last n data points.
- Weighted Moving Average: Assigns different weights to data points, giving more importance to recent observations.
- Exponential Smoothing: Assigns exponentially decreasing weights to past observations. Useful for capturing trends and seasonality.
- Simple Exponential Smoothing: Suitable for data without trend or seasonality.
- Holt-Winters Exponential Smoothing: Handles data with both trend and seasonality.
- ARIMA Models: Combines autoregressive (AR) and moving average (MA) components, along with differencing (I) to stabilize the series.
- AR (Autoregressive): Uses past values to predict future values.
- MA (Moving Average): Uses past forecast errors for prediction.
- Integrated (I): Involves differencing the data to make it stationary.
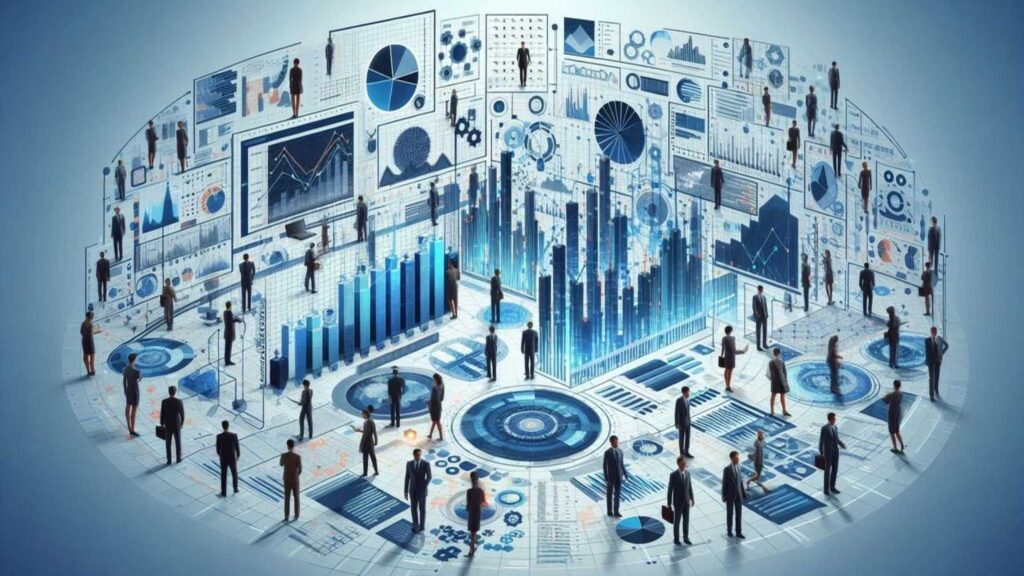
Understanding the key components of time series data, including trend, seasonality, cycles, and irregularities, is crucial for students to effectively analyze and forecast temporal patterns. Techniques such as moving averages, exponential smoothing, and ARIMA models enable students to smooth out fluctuations, capture trends and seasonality, and make accurate predictions, equipping them with the necessary skills to analyze and interpret time-dependent data in various domains.
Statistical Software and Tools
Statistical software and tools, such as R, Python, SPSS, and Excel, provide students with powerful platforms for data analysis, visualization, and modeling. These tools offer a wide range of statistical functions, packages, and libraries, allowing students to efficiently manage, analyze, and interpret complex datasets.
Overview of Popular Statistical Software Packages
Several statistical software packages are widely used in time series analysis, offering a range of tools for data manipulation, modeling, and visualization. Each software has unique features and capabilities that cater to different analytical needs.
- SPSS: User-friendly interface with strong capabilities for statistical analysis and modeling, including time series analysis.
- SAS: Comprehensive suite for advanced analytics, data management, and predictive modeling.
- R: Open-source software with extensive libraries for statistical analysis and visualization.
- Python: Versatile programming language with powerful libraries such as pandas, statsmodels, and sci-kit-learn for time series analysis.
Features and Capabilities of Each Software/Tool
Each software/tool provides distinct features and capabilities that make them suitable for various aspects of time series analysis.
- SPSS:
- Intuitive GUI for easy data handling and analysis.
- Extensive options for time series modeling and forecasting.
- Integration with other IBM analytics tools.
- SAS:
- Robust data manipulation and advanced analytics capabilities.
- Strong support for time series analysis with procedures like PROC ARIMA, and PROC TIMESERIES.
- Scalability for large datasets and enterprise-level applications.
- R:
- Comprehensive libraries such as forecast, series, and texts.
- Flexibility in data manipulation and advanced statistical modeling.
- Strong visualization support with ggplot2 and plotly.
- Python:
- Libraries like pandas for data manipulation, statsmodels for statistical modeling, and sci-kit-learn for machine learning.
- Integration with Jupyter Notebooks for interactive analysis and visualization.
- Wide range of visualization tools including matplotlib, seaborn, and Plotly.
Statistical software packages like SPSS, SAS, R, and Python provide students with a diverse set of tools and capabilities for time series analysis, offering intuitive interfaces, robust data manipulation features, advanced modeling techniques, and powerful visualization options to help them effectively analyze, interpret, and communicate insights from temporal data across various domains.
Data Visualization for Statistical Analysis
Data visualization plays a crucial role in statistical analysis by providing intuitive and informative representations of complex data. Techniques like scatter plots, histograms, box plots, and time series plots help students explore patterns, identify outliers, and communicate findings effectively.
Importance of Data Visualization in Statistical Analysis
Data visualization is crucial in statistical analysis as it helps in understanding data patterns, trends, and relationships. Effective visualizations can simplify complex data, making it easier to communicate insights and support decision-making.
- Understanding Patterns: Visualizations help identify trends, seasonal patterns, and anomalies in the data.
- Simplifying Complex Data: Graphical representations make complex data more accessible and understandable.
- Communicating Insights: Visuals are essential for presenting findings to stakeholders clearly and effectively.
Types of Visualizations Suitable for Different Types of Data and Analyses
Different types of data and analyses require specific visualization techniques to effectively convey the underlying information.
- Line Charts: Ideal for time series data to show trends over time.
- Bar Charts: Suitable for comparing categorical data.
- Histograms: Used for showing the distribution of a single variable.
- Scatter Plots: Effective for visualizing relationships between two variables.
- Box Plots: Useful for displaying the distribution and identifying outliers.
- Heatmaps: Great for showing correlations and patterns in large datasets.
- Pie Charts: Best for illustrating proportions within a whole, though limited in conveying complex information.
Data visualization is a crucial component of statistical analysis, as it helps students understand patterns, trends, and relationships within complex datasets, while also simplifying the communication of insights to stakeholders. Techniques such as line charts, bar charts, histograms, scatter plots, box plots, and heatmaps enable students to effectively explore, analyze, and present their findings, enhancing their ability to make informed decisions based on the data.
Statistical Tools and Techniques: Applications Explored
Statistical tools and techniques, such as regression analysis, ANOVA, time series forecasting, and multivariate analysis, have a wide range of applications across diverse fields, including business, healthcare, social sciences, and engineering, empowering students to tackle complex problems and make data-driven decisions.
Real-World Examples and Case Studies
Statistical methods are applied across various domains to extract meaningful insights from data. Examples include predicting patient outcomes in healthcare, optimizing financial portfolios in finance, and targeting customer segments in marketing.
- Healthcare: Predicting patient readmission rates using logistic regression models.
- Finance: Forecasting stock prices with time series analysis and ARIMA models.
- Marketing: Segmenting customers based on demographic and behavioral data using clustering algorithms.
Applications Across Various Industries
Statistical techniques are pivotal in decision-making across industries, ensuring informed strategies and operational efficiencies. Healthcare relies on statistical analysis for clinical trials and epidemiological studies, while finance utilizes risk modeling and fraud detection algorithms. Marketing employs A/B testing and predictive analytics for campaign optimization.
- Healthcare: Analyzing clinical trial data to assess drug efficacy and safety.
- Finance: Using regression analysis to model factors influencing loan default rates.
- Marketing: Applying sentiment analysis on social media data to gauge brand perception.
Real-world examples and case studies demonstrate the practical applications of statistical methods across diverse domains, empowering students to understand how these techniques can be leveraged to extract meaningful insights, optimize decision-making, and drive innovation in fields such as healthcare, finance, and marketing. By exploring case studies that highlight the use of statistical analysis in predicting patient outcomes, forecasting stock prices, segmenting customers, and optimizing marketing campaigns, students gain a deeper appreciation for the versatility and impact of statistical methods in solving real-world problems.
Ethical Considerations in Statistical Analysis
Ethical considerations are paramount in statistical analysis, ensuring the responsible and transparent use of data. Key aspects include data privacy, informed consent, bias mitigation, and accurate reporting of findings, enabling students to conduct research with integrity and respect for human subjects and stakeholders.
Data Privacy and Confidentiality
Maintaining data privacy is critical in statistical analysis to protect individuals’ sensitive information. Encryption, anonymization techniques, and secure data storage practices are employed to prevent unauthorized access and breaches.
- Encryption: Using cryptographic techniques to secure data during transmission and storage.
- Anonymization: Removing or obfuscating personally identifiable information (PII) from datasets.
- Access Controls: Implementing strict access controls and authentication mechanisms to limit data access to authorized personnel only.
Bias and Fairness in Statistical Modeling
Addressing bias is essential to ensure fairness in statistical models. Techniques like balanced sampling, fairness-aware algorithms, and regular audits help mitigate biases that could lead to skewed or discriminatory outcomes.
- Balanced Sampling: Ensuring representation from diverse groups to avoid underrepresented biases.
- Fairness-Aware Algorithms: Adjusting model outputs to minimize disparate impacts on different demographic groups.
- Audits and Reviews: Regularly reviewing model performance and outcomes to detect and correct biases.
Compliance with Ethical Guidelines and Regulations
Adhering to ethical guidelines and regulatory frameworks such as GDPR and HIPAA is imperative in statistical analysis. This involves obtaining informed consent, transparent data usage policies, and adhering to legal standards to protect individuals’ rights and ensure ethical conduct.
- Informed Consent: Obtaining explicit permission from participants before collecting and using their data.
- Transparent Policies: Communicating data usage practices and potential risks to stakeholders.
- Regulatory Compliance: Adhering to legal frameworks and guidelines specific to data privacy and protection.
Ethical considerations are crucial for students conducting statistical analysis, as they must ensure the responsible and transparent use of data.
This includes maintaining data privacy and confidentiality through encryption, anonymization, and access controls, addressing bias and fairness in statistical modeling, and complying with ethical guidelines and regulations, such as obtaining informed consent and adhering to data privacy laws.
Upholding these ethical principles enables students to build trust, protect individual rights, and produce reliable and unbiased findings.
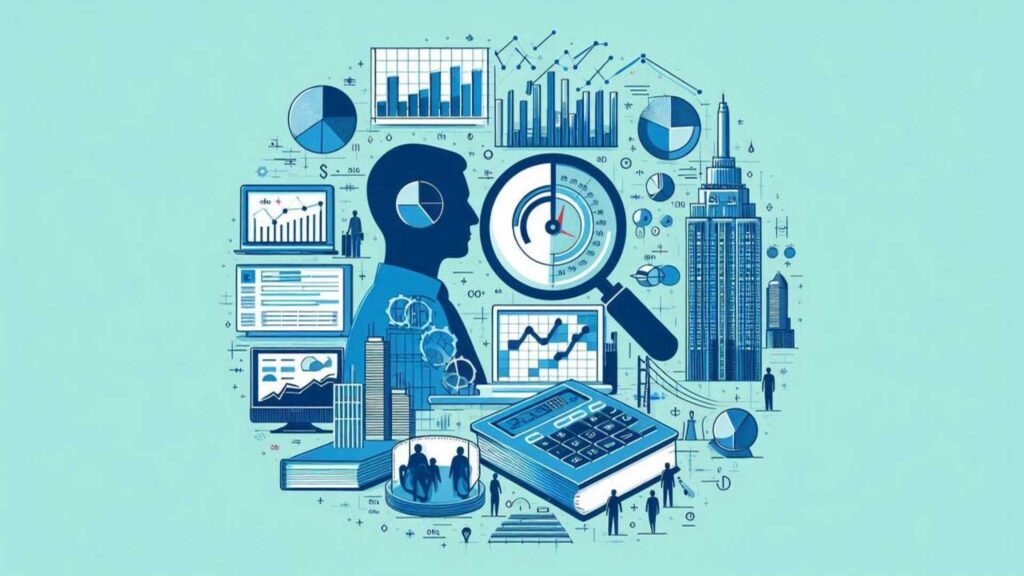
Conclusion
Statistical tools and techniques encompass a wide array of methods essential for analyzing and interpreting data. Key techniques include regression analysis, hypothesis testing, machine learning algorithms, and time series analysis, each serving distinct purposes in extracting insights from data.
- Regression Analysis: Modeling relationships between variables to understand dependencies.
- Hypothesis Testing: Evaluating the significance of observed effects or differences.
- Machine Learning Algorithms: Utilizing algorithms like decision trees, neural networks, and clustering for predictive analytics.
- Time Series Analysis: Forecasting future trends based on historical data patterns.
Future Trends and Advancements in Statistical Analysis
The future of statistical analysis is shaped by advancements in machine learning, big data analytics, and AI-driven methodologies. Trends include the integration of AI for automated decision-making, enhanced interpretability of complex models, and the democratization of analytics tools for broader accessibility and usability.
- AI and Automation: Leveraging AI to automate model development, optimization, and deployment processes.
- Interpretability: Improving the transparency and interpretability of black-box models like neural networks.
- Democratization: Making advanced statistical tools and techniques accessible to non-experts through user-friendly interfaces and cloud-based platforms.
To seize this opportunity, we need a program that empowers the current IT student community with essential fundamentals in data science, providing them with industry-ready skills aligned with their academic pursuits at an affordable cost. A self-paced program with a flexible approach will ensure they become job-ready by the time they graduate. Trizula’s Mastery in Data Science is the perfect fit for aspiring professionals. It equips them with the necessary fundamentals in contemporary technologies such as data science, laying the groundwork for advanced fields like AI, ML, NLP, and deep science. Our program is committed to equipping engineering students with marketable skills that will serve as a solid foundation for future professional advancement. click here to get started!
FAQs:
1. What are the statistical techniques used in data science?
Some of the most important statistical techniques used in data science include linear regression, classification, resampling methods, subset selection, shrinkage, dimension reduction, nonlinear models, tree-based methods, support vector machines, and unsupervised learning techniques like principal component analysis and clustering.
2. What are the statistical tools and techniques for data analysis?
Key statistical tools and techniques for data analysis include descriptive statistics (measures of central tendency and dispersion), inferential statistics (hypothesis testing, confidence intervals), regression analysis, ANOVA, correlation analysis, experimental design, and multivariate techniques like PCA and factor analysis.
3. What are the 5 most important methods for statistical data analysis?
The 5 most widely used statistical data analysis methods are:
- Mean (average)
- Standard deviation
- Regression analysis
- Hypothesis testing
- Sample size determination
4. What is a statistical technique?
A statistical technique is a method or approach used to collect, analyze, interpret, and present data to uncover patterns, trends, and relationships. Statistical techniques rely on probability theory and mathematical principles to draw inferences and make decisions based on data.
5. What are the techniques of data science?
Key techniques used in data science include data collection and preprocessing, exploratory data analysis, statistical modeling, machine learning algorithms (supervised and unsupervised), deep learning, natural language processing, computer vision, and data visualization. Data science combines programming, statistics, and domain expertise to extract insights and knowledge from structured and unstructured data